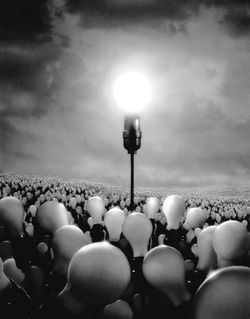
What does the term “peer effects” mean in a school environment? It includes the effects of students’ teaching one another, but that is only the most direct form of peer effects. Intelligent, hard-working students can affect their peers through knowledge spillovers and through their influence on academic and disciplinary standards in the classroom. Alternatively, misbehaving students may disrupt the classroom, thereby sapping their teacher’s time and energy. The makeup of a classroom-its average family income, the number of children with disabilities, its racial and gender balance-can also create peer effects. Children with learning disabilities may draw disproportionately on their teacher’s time; racial or gender tension in the classroom may interfere with learning; wealthier parents may purchase learning resources that get spread over a classroom. Peer effects may even operate through the ways in which teachers or administrators react to students. For instance, if teachers believe that less should be expected of minority children, they might lower their academic standards when confronted with a classroom that has a high share of black or Hispanic students. The other students in such a classroom would experience negative peer effects, not due to the minority students’ influence but because of the teacher’s assumptions.
Peer effects, if they do indeed exist, have implications for a number of policy issues in education. For example, the literature on school finance and control is currently absorbed with the question of whether students are affected by the achievement of their schoolmates. If peer effects exist at school, a school-finance system that encourages an efficient distribution of peers among all schools will make society’s investments in student learning more productive. The debate over “tracking,” the system in which students are exposed only to peers with similar achievement, turns partly on the question of whether being concentrated in lower-level classrooms merely exacerbates the problems of low-achieving children. Desegregation plans that assign students to schools outside their neighborhood or school district also rest partly on the belief that one’s peers can exercise enormous influence over one’s performance.
However, there are two principal difficulties for theories that rely on peer effects. First, however much sense the theory of peer effects makes, there are formidable obstacles to estimating them. Although some credible estimates of peer effects do exist, people often rely on evidence that is seriously biased by selection effects. For instance, if everyone in a group is high achieving, many observers assume that achievement is an effect of belonging to the group instead of a reason for belonging to it.
Also, the most popular model used by researchers to estimate peer effects (the “baseline” model) assumes that peer effects are a zero-sum phenomenon-that is, in order to give one student a better peer, that peer must be taken away from another student; the two effects cancel one another out. According to the baseline model, a student’s reading score would be affected linearly by the average reading score of his classmates. Regardless of how one allocates peers, total societal achievement remains the same under the baseline model. But many arguments assume that total societal achievement can be increased if peers are redistributed. For instance, the argument against “tracking” is based on the notion that both low- and high-achieving students benefit from being exposed to one another in the classroom. By contrast, the idea behind “gifted and talented” programs is that high-achieving students benefit from being among one another. Thus, although it is tempting to dismiss the baseline model as naive or restrictive, if one were able to show, empirically, that the baseline model adequately described peer effects, some interesting theories would fall by the wayside.
The central problem with estimating peer effects in schools is that families, in a number of ways, can select their children’s peers. Families self-select into schools based on their incomes, job locations, residential preferences, and educational preferences. A family may even self-select into a school based on the ability of an individual child; a family with a highly able child may choose to live near a school that has a program for gifted children. Moreover, families may influence the particular classes to which their children are assigned within their schools. If, for example, savvy parents believe that a certain 3rd grade teacher is particularly good, they may get their children assigned to her class, thereby creating a classroom of children whose parents care about education to an unusual degree. School administrators and teachers can also select students into particular classrooms for reasons that are related to achievement. For instance, a school may assign children with similar achievement levels to the same classroom, in order to minimize teaching difficulty. Or a school may place all of the “problem” students in a certain teacher’s class because she is good at dealing with them. In short, it should be assumed that a child’s being in a certain school, and even a particular classroom, is associated with unobserved variables-such as highly involved parents-that affect his achievement.
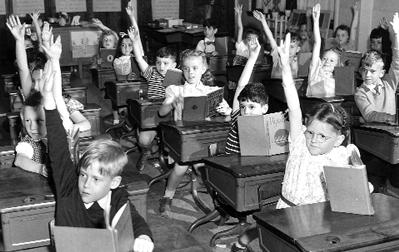
New Strategies
This study introduces two empirical strategies that circumvent these obstacles by examining differences in cohorts of students-a school’s group of 3rd graders in one year versus the next year’s group of 3rd graders-rather than cross-sections of classrooms at the same grade level. Both strategies depend on the idea that the peer composition of a certain grade within a school-its gender and racial balance, its mix of the studious and the troublesome-will vary from year to year in a way that is idiosyncratic and beyond the easy management of parents and schools. Even within a school that has an entirely stable population of families, timing and simple biological variation would create birth cohorts that idiosyncratically vary in their natural talents and racial and gender makeup.
Suppose, for instance, that a family shows up for kindergarten with their older son and finds that, simply because of random variation in local births, their son’s cohort is 80 percent female. The next year, they show up with their younger son and find that, also because of random variation, his cohort is 30 percent female. Their older son will be exposed to more female students (who tend to be higher achievers in elementary school). Their younger son will be exposed to more male students. Because the two boys have the same parents and the same school, the main difference in their experience will be their peers.
It is this type of unexpected variation in cohort composition that the empirical strategies in this study attempt to exploit. A parent may have a fairly accurate impression of the cohorts around his child’s age, and may pick a school on that basis, but it is difficult for a parent to react to a cohort composition “surprise” by changing schools. As long as we focus on idiosyncratic variation in cohort composition, as opposed to classroom composition, we need not worry about how schools and parents manipulate the assignment of students to classrooms. If a cohort is more female than the previous cohort, for instance, the school must allocate the “extra” females among its classrooms somehow. Inevitably, some students in the cohort will end up with a peer group that is more female than is typical.
In the first strategy, I attempt to identify idiosyncratic variation in cohort composition by comparing adjacent cohorts’ gender and racial makeup. I see whether differences in the achievement of adjacent cohorts within a certain grade within a certain school are systematically associated with differences in the gender composition of those cohorts. If there are no peer effects, the average achievement of male (or female) students should not be affected by the share of their peers who are female.
In the second strategy, I attempt to identify the idiosyncratic component of each gender and racial group’s achievement and determine whether the components are related to one another. For instance, if the females in the 1996-97 cohort of 3rd graders in School I have unusually low achievement, does one find that the males in the 1996-97 cohort of 3rd graders in School I have unusually low achievement too? If the Hispanic students in the 1994-95 cohort of 5th graders in School II have unusually high achievement, does one find that the white, black, and Asian students in the 1994-95 cohort of 5th graders in School II have unusually high achievement too?
This strategy requires an unbiased estimate of the idiosyncratic component of each group’s achievement that is independent of the estimates with which one plans to correlate it-that is, the idiosyncratic component of the average achievement of other groups within the same cohort. This is accomplished by using only that portion of the achievement of a gender or racial group that cannot be explained by a linear time trend and the overall gender and racial composition of the group’s cohort.
For both strategies, I am sensitive to the potential criticism that what appears to be idiosyncratic variation in racial groups’ shares or achievement may actually be a time trend within a grade within a school. To address this criticism, I eliminate not only linear time trends but also any school in which actual years explain more variation (in cohort composition or in achievement) than false, randomly assigned years. These empirical strategies are, I would argue, an improvement on previous methods of identifying peer effects in schools. Previous researchers have most often estimated models like the baseline model and used cross-sectional variation in schoolmates to identify peer effects. They have dealt with the problem of selection bias by controlling for observable variables, comparing the educational experiences of siblings in families that have moved (so that the siblings experience different schools), studying children in magnet or desegregation programs, or estimating a selection model. In particular, Boston’s Metco program, in which inner-city minority children are sent to schools in the suburbs, has been much studied. The difficulty with estimates based on programs like Metco is that children who enter the program (and do not leave it) are likely to have higher unobserved ability or motivation. In practice, these methods have generally proved unconvincing because there are unobservable variables that are correlated with peer selection, with moving, with participating in a magnet or other school program, or with the excluded variables that identify the selection model.
Only in some cases am I able to distinguish among the various channels through which peer effects can operate. In general, the peer effects estimated in this study (and in most research) embody multiple channels. In judging the magnitude of the results, it is important to keep the multiple channels in mind.
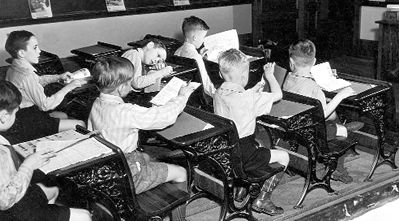
Data
Data for the entire population of 3rd, 4th, 5th, and 6th graders in public schools in the state of Texas during the 1990s were used. Beginning with the 1990-91 school year, Texas began to administer a statewide achievement test, the Texas Assessment of Academic Skills (TAAS), to elementary-school students. Scores on the TAAS form the basis of the analysis. Texas contains a very large number of elementary schools, which is fortunate because idiosyncratic variation in the gender and racial makeup of cohorts within a grade within a school is sufficiently uncommon that a large number of observations are needed to generate the necessary number of “natural events.”
In a typical year during the 1990-91 to 1998-99 period, there were about 3,300 schools in Texas that enrolled 3rd graders; the size of the median cohort was about 80 students. Third graders were typically 49 percent female, 0.3 percent Native American, 2 percent Asian, 15 percent black, 33 percent Hispanic, and 49 percent Anglo. There were no apparent time trends in the shares of 3rd graders who were female or Native American. There were slight upward trends in the shares of third graders who were Asian (2.2 to 2.5 percent over the period), black (14.8 to 15.7 percent over the period), and Hispanic (30.7 to 34.9 percent). There was a mild downward trend in the share of 3rd graders who were Anglo (52.2 to 46.4 percent). The statistics for grades 4, 5, and 6 were very similar (naturally, because most of the students remain in these schools from year to year).
Young girls tend to be better readers than young boys. On the 3rd grade reading test, the average female scored 1.1 points-about half a standard deviation-higher than the average male. Some ethnic differences were even larger. Compared with the average white student, the average black student scored 3.6 points lower; the average Hispanic student, 2.9 points lower; the average Asian student, 0.7 points higher; and the average Native American student, 1.5 points lower. The black-white and Hispanic-white score gaps are substantial: 1.6 and 1.3 standard deviations, respectively.
There was an upward trend in the reading scores of all groups over the period, the average score rising from 28.5 to 31.3 points. Some improvement typically occurs during the first few years of administering a new test, simply owing to comfort with the test. The improvement in Texas accelerated over time, however, and the past few years’ improvement are most likely due to true learning of the material tested by the examinations-particularly as Texas’s curriculum and tests became more closely aligned.
There was a slight upward trend in math scores as well: an average gain of 0.1 points per year. The average female scored 0.1 points higher than the average male-a difference of only 0.03 standard deviations. Compared with the average white student, the average black student scored 4.7 points lower; the average Hispanic student, 3.2 points lower; the average Asian student, 1.3 points higher; and the average Native American student, 1.9 points lower. The black-white and Hispanic-white score gaps are again substantial: 1.6 and 1.1 standard deviations, respectively. The results on the 4th, 5th, and 6th grade tests were very similar to those in 3rd grade.
Results of Strategy 1
Gender. Both boys and girls tend to perform better in reading when they are in classes with larger shares of girls (see Figure 1). For instance, in 3rd grade reading, girls’ scores rise by 0.037 points for every 10 percentage point change in the share of their class that is female. Males’ scores rise by 0.047 points for every 10 percentage point change in the share of their class that is female. To put this in perspective, an all-female class would score about one-fifth of a standard deviation higher in reading, all else being equal. The effects for 4th, 5th, and 6th grade reading scores are similar. A translation of the results in a way that reveals the effects of peer achievement provides a different perspective: being surrounded by peers who score 1 point higher on average raises a student’s own score by 0.3 to 0.5 points, depending on the grade. The translation suggests that peer effects are substantial.
Boys and girls also perform better in math when they are in classes with larger shares of girls. In 3rd grade reading, girls’ scores rise by 0.038 points for every 10 percentage point change in the share of their class that is female. The effect is larger in higher grades: female 6th graders’ scores rise by 0.064 points for every 10 percentage point change in the share of their class that is female. Likewise, male 3rd graders score 0.040 points higher and male 6th graders score 0.081 points higher for every 10 percentage point change in the share of their class that is female. Because the average female scores only a little higher than the average male, however, the earlier translation of the scores generates implausibly large effects. If the translated effects were taken literally, one would conclude that being surrounded by peers whose math scores were on average 1 point higher would raise a student’s own score by 1.7 to 6.8 points, depending on the grade. These effects are so large that they suggest that peer effects do not operate purely through the channel of peers’ achievement in math.
There are a few alternative channels that might explain the effect of females on math scores. First, since learning math requires reading, and reading scores are higher in classes with higher percentages of females, females may affect subjects like math through their (quite plausible) peer effect on reading. Second, classes with more girls may simply have fewer disruptive students or a more learning-oriented culture. Third, classroom observers have argued that the pressure to be feminine makes girls unenthusiastic about math. Perhaps in female-dominated classrooms, girls do not experience this kind of pressure and therefore remain enthusiastic about math-thereby allowing the teacher to teach it better to all students. In any case, it is clear that the baseline model of peer effects is inadequate: peer effects do not operate solely through peers’ mean achievement in the same subject.
Race. In interpreting the next set of results, it is worthwhile to remember that the peer effects of any racial group include the effect of variables associated with that group, including their family income, parents’ education and level of involvement, and the language spoken in the home. They should not be interpreted as the effects of a group’s innate ability. In particular, black and Hispanic students are far more likely to be poor than are white students in Texas. Therefore, any negative peer effects associated with being in classes with large shares of black students largely reflect the impact of being exposed to low-income students.
Black, Hispanic, and white 3rd graders all tend to perform worse in reading and math when they are in classes that have a larger share of black students. For every 10 percentage point rise in the share of their class that is black, black students’ reading scores fall by 0.250 points, Hispanic students’ reading scores fall by 0.098 points, and white students’ reading scores fall by 0.062 points. For the same 10 percentage point change in the share of their class that is black, black students’ math scores fall by 0.186 points, Hispanic students’ math scores fall by 0.086 points, and white students’ reading scores fall by 0.043 points. What’s particularly interesting is that having more black peers appears to be most damaging to other black students. Recalling that black students have the lowest scores on both the reading and math tests, one can see that these results can be interpreted as the effects of peer achievement. A translation of the results shows that being surrounded by peers who score 1 point lower on average has the following effects: it lowers a black student’s own score by 0.676 points in reading and 0.402 points in math; it lowers a Hispanic student’s own score by 0.266 points in reading and 0.185 points in math; and it lowers a white student’s own score by 0.168 points in reading and 0.092 points in math. The translation suggests that the effect of average peer achievement varies from small (0.092) to substantial (0.676) and that average peer achievement has its most substantial effects within racial groups.
In the 4th, 5th, and 6th grades only, Hispanic students perform worse in reading and math and white students perform worse in math when they are in classes with a larger share of Hispanic students. For instance, for every 10 percentage point rise in the share of their class that is Hispanic, Hispanic 5th graders’ reading scores fall by 0.142 points and their math scores fall by 0.205 points. With the same change in the Hispanic share, white 5th graders’ math scores fall by 0.061 points. A translation of the results finds that being surrounded by peers who score 1 point lower on average has the following effects: it lowers a Hispanic student’s own score by 0.439 points in reading and 0.587 points in math, and it lowers a white student’s own score by 0.176 points in math. Again, the results suggest that the effects of average peer achievement vary and are greatest for peers who are within the racial group that is generating the change in achievement.
There were a few results for Asian students that were statistically significant. Each of these results showed Asian students’ having positive peer effects in math. For instance, with every 10 percentage point increase in the share of their class that is Asian, white 5th graders’ math scores rise by 0.072 points and white 6th graders’ math scores rise by 0.202 points. This comports with the interpretation that average peer achievement influences everyone’s test scores, since Asians score higher than whites in math overall (the Asian-white score gap is positive and relatively large in math, 0.62 of a standard deviation in the 4th, 5th, and 6th grades).
The fact that peer effects appear to be stronger for members of the same race or ethnicity than across racial and ethnic groups suggests that the baseline model, in which the average achievement of one’s peers has a linear effect on one’s own achievement, is inadequate. Let’s further explore the question of nonlinear peer effects by examining whether peer effects are different at various starting points: when the initial cohort is 0 to 33 percent black, 33 to 66 percent black, or 66 to 100 percent black.
Three patterns stand out. First, the negative peer effect of black students on black students’ own scores is largest in cohorts that are between 33 and 66 percent black. The negative effect of black students on white students’ own scores is largest in cohorts that are at least 33 percent black. I performed the same test with Hispanic students. The negative effect of Hispanic students on Hispanic students’ own scores only appears in cohorts that are 0 to 33 percent Hispanic. In fact, Hispanic students have a statistically significant, positive effect on the achievement of Hispanic students in cohorts that are 66 to 100 percent Hispanic (see Figure 2).
There are a few possible interpretations of this last finding. First, having even more Hispanic peers in a cohort that is already mainly Hispanic may be helpful because each student who has difficulty speaking English is more likely to find a bilingual student to translate for him, to help him learn English, and so on. Second, an overwhelmingly Hispanic cohort may be helpful because it makes teachers sensitive to providing instruction that can be understood by students with limited English proficiency. Third, some schools, when faced with an unusually large Hispanic cohort, may segregate their Spanish-speaking students in a particular classroom because there are enough students to fill such a class. It is possible that such segregation generates higher achievement among Hispanic students (even if it is undesirable for other reasons). Regardless, this finding clearly shows that peer effects do not operate only in a linear fashion.
Results of Strategy 2
Remember that Strategy 2 uses cross-sectional data to study the impact on other groups when a particular gender or racial group experiences unusually high or low achievement. In the gender comparison, all of the results show that one gender’s idiosyncratic achievement has a positive, highly statistically significant effect on the idiosyncratic achievement of its peers from the other gender group. In grades 3 through 6, being surrounded by peers who score one point higher in reading raises a student’s own score by 0.3 to 0.4 points. Being surrounded by peers who score one point higher raises a 3rd grader’s own math score by about 0.6 points, a 4th grader’s own score by about 0.5 points, and a 5th or 6th grader’s own score by about 0.4 points. In the racial and ethnic comparison, the results show that being surrounded by peers who score 1 point higher in reading raises a student’s own reading score by 0.3 to 0.8 points. In general, the math results were similar to the results for reading.
In short, Strategy 2 generates unambiguous evidence about the existence of peer effects, but the range of estimates is somewhat wide: 0.10 to 0.55 points is a plausible summary of the range, given the various results and known biases.
Conclusion
The peer effect estimates generated by the two strategies are reasonably similar. Strategy 1 found that being surrounded by peers who score 1 point higher raises a student’s own score between 0.15 and 0.40 points. Strategy 2 tends to estimate an increase of between 0.10 and 0.55 points when a student is surrounded by peers who score 1 point higher. These estimates confirm that peers’ ability levels affect achievement in ways that policymakers and researchers should not ignore.
Both strategies also showed that the baseline model of linear peer effects is inadequate. My results provide little evidence of general asymmetry, such as low achievers gaining more by being with high achievers than the amount high achievers lose by being with low achievers. However, I do show that peer achievement is not the sole channel for peer effects. The large, positive effect that a prevalence of girls has on boys’ math scores cannot plausibly be explained solely by girls’ effect on average peer achievement in math. Likewise, I found that a rising share of Hispanics has a positive effect on certain Hispanic students’ scores, which could not be an effect of average peer achievement since raising the Hispanic share lowers average peer achievement. In addition, some results suggest that peer effects are stronger inside racial groups than between racial groups.
Caroline M. Hoxby is a professor of economics at Harvard University and a visiting fellow at the Hoover Institution, Stanford University. The unabridged version of this article is available at www.educationnext.org