This article is part of a new Education Next series commemorating the 50th anniversary of James S. Coleman’s groundbreaking report, “Equality of Educational Opportunity.” The full series will appear in the Spring 2016 issue of Education Next.
When I reflect on James Coleman and the “Equality of Educational Opportunity”study (EEOS), I am immediately inclined to quote Ecclesiasticus 44:1: “Let us now praise famous men, and our fathers that begat us.” Coleman is the father of much social scientific analysis of education. There is a great deal to admire in his work, and, thus, this essay begins in praise. Remember that, reader, because—though I might wish otherwise—it cannot continue in a strain of unmitigated praise throughout. First the praise, however.
Fifty years on, the sheer scale and thoroughness of the EEOS remain mind-boggling. Despite all of today’s talk of “big data,” there is no contemporary survey-based data set of education that is on a comparable scale to the EEOS. It dwarfs recent surveys conducted by the National Center for Education Statistics (NCES). The workhorse version of the EEOS contains 567,148 students, 44,193 teachers, and 3,941 principals. In comparison, today’s Education Longitudinal Study comprises only about 15,000 students in 750 schools. The accomplishment that the EEOS represents remains immense. Perhaps 1960s scholars had no sense of limits and thus conquered all? Perhaps 1960s survey respondents were incredibly cooperative? In any case, if Coleman were to return in ghostly form and ask me to lead such a study today, I would certainly light all the lamps in the hope of dispelling his apparition.
Prior to the EEOS, government agencies gathered data, but they used it almost exclusively to publish aggregate statistics. The EEOS aspired to analyze student data in a deeper way, by correlating achievement and other factors at an individual level. In so doing, it illuminated a world of student heterogeneity that had remained obscure until then. It is not merely that the EEOS data were subsequently used in a great deal of research; it is that such research and much research based on other data would have remained unimaginable without the EEOS. Here endeth the first note of praise.
I am too young to have known Coleman personally as a scholar, but I have heard from numerous scholars who did know him that he commenced the EEOS thinking that it would demonstrate, among other things, the importance of school inputs: teacher characteristics, class size, and the like. Of course, it did nothing of the sort. Yet, despite my arguing below that much of Coleman’s analysis was not only wrong but generated misunderstandings that remain sadly pervasive today among naive scholars, I admire Coleman’s courage and empiricism. Given his understanding of statistics and social scientific analysis, he let his conclusions accurately reflect what he believed he had found. About how many social scientists can we say something comparable? So many scholars enter studies with preconceived notions and force those same notions out at the exit, allowing no room in between for true empiricism to alter the preordained conclusions. Coleman was a true social scientist for his day, and for more than that we cannot ask. Here endeth the second note of praise.
A Flawed Analysis
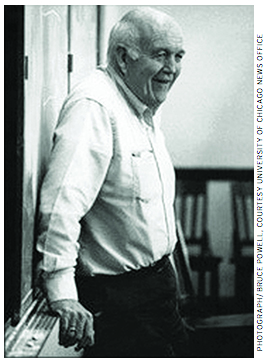
Coleman did nearly all of his work before the advent of the causal revolution in social science and seems not to have anticipated it. Like many other statistically minded social scientists of his time, he thought of regression and analysis of variance as tools that did not merely break an outcome, such as achievement, into partial correlations. He thought these tools generated coefficients that magically represented causal effects. Thus, although Coleman’s descriptions of data may be praiseworthy, his analysis and interpretation are wrong. More precisely, although his conclusions may be correct (some are, in my estimation), they are unwarranted based on his research.
It is worthwhile elaborating on the last point. The problem with Coleman’s work and much of the work spawned by the EEOS is not that it necessarily came to the wrong conclusions, but that the methods were so flawed that the conclusions were unjustified. It is far more intellectually damaging to scholarship to push a conclusion that happens to be right but is unwarranted based on the research than it is to push a conclusion that is wrong but is based on sound methods and that went astray for some extraordinary reason (such as data that were accidentally jumbled without the researcher’s knowledge). The latter type of error is easily corrected by other later scholars, who adopt sound methods. The former type of error produces scholarly and intellectual confusion for decades, as Coleman did, and to some extent still does.
To see the flaws in Coleman’s reasoning, consider an archetypal analysis in the style of the EEOS and the studies it spawned. It is a regression in which student achievement is explained by a combination of school inputs (resources such as funding per student, class size, teacher qualifications, etc.) and the characteristics of peers (percentage of schoolmates who are white and who are black, etc.), families (race, ethnicity, parents’ education, number of siblings, etc.), and neighborhoods (the share of households who rent versus own, etc.). Coleman found that family and peer characteristics explained a statistically and consequentially significant amount of variation in the measure of achievement. School inputs and neighborhood characteristics did so to a much lesser extent. So far as an analysis of variance goes, all this is correct. But Coleman then concluded that families and peers had an effect on achievement that schools and neighborhoods did not.
Coleman’s conclusion was wholly unjustified, because little or none of the EEOS variation in families, schools, peers, or neighborhoods came from true experiments, policy experiments, natural experiments, or any other plausibly exogenous source. If the EEOS had wanted to draw conclusions about the effects of families, schools, peers, and neighborhoods, it would have needed to conduct or locate experiments for each variable whose effect it wanted to identify. For instance, to identify the effect of family income, researchers need an experiment in which some families are arbitrarily given an income shock (as in the Income Maintenance Experiments of 1968 through 1979). To identify the effect of school spending, they need credibly exogenous variation in districts’ funding (such as sometimes arises through quirks in a state’s school-finance formula). To identify the effects of peers, they need to find situations in which students have been arbitrarily or randomly shifted among classrooms or schools. For example, Gretchen Weingarth and I, in “Taking Race Out of the Equation: School Reassignment and the Structure of Peer Effects,” exploit random variation in school assignments generated by Wake County’s efforts to keep its schools similarly diverse on racial and economic grounds. To identify the effect of neighborhoods, researchers need something like the Moving to Opportunity experiment, in which randomly selected low-income households were induced to reside in different neighborhoods.
The Wrong Takeaways
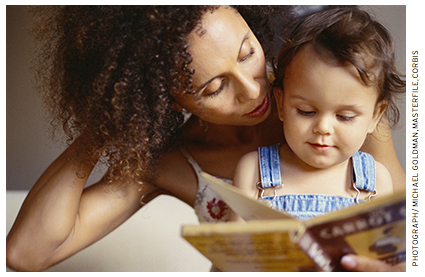
It would have been very difficult for the EEOS to satisfy all of these needs at the same time, but this is what would have been necessary to justify its conclusions. In retrospect, it is painfully obvious that much of the debate touched off by the Coleman Report was not necessary at all. Scholars were debating conclusions that were unwarranted in the first place, and their debates were often as uninformed by causal methods as the EEOS conclusions.
To see how problematic Coleman’s lack of differentiation between causality and correlation was, consider one of the most often cited EEOS “takeaways”: families matter a great deal, and schools do not. Given its analysis, the Coleman Report should have concluded only that family characteristics explain achievement through
1) direct channels (for instance, the educated parent reads to her children in a more instructive manner);
2) the indirect channel that works through families’ choices of schools, in which the school characteristics relevant to achievement are more fully captured by what parents observe than by the short list of school descriptors in the regression (for instance, well-educated parents choose teachers with higher value-added); or
3) other indirect channels that work through families’ choices of neighborhoods, extracurricular activities, religious participation, and so on.
Since people tend to become confused about the direct and indirect channels and how they relate to a regression, it is worthwhile elaborating on the statements in the previous paragraph. First, why is an indirect channel that works through family choices not equivalent to the direct channel? (That is, if something is the result of family choices, how is this different from saying that it is something that the family itself does?) The indirect channels are different because, if we shut down the choices, families would be unable to generate the same effects on their own. For instance, suppose that our society disallowed schools with a sound curriculum (as China arguably did during the Cultural Revolution). Or, suppose that there were no teachers with high value-added because selection or preparation of teachers was extremely poor. Then, regardless of how advantaged parents were, they would find it hard to generate high achievement in their children. That is, it is very difficult for good parents to be good if we preclude their access to good schools and teachers. Better parents can make better choices only if those choices are available to them.
Second, why did I specify school characteristics “relevant to achievement [that] are more fully captured by what parents observe than by the short list of school descriptors”? Regressions only operate with the measures we give them, and these measures are typically crude and erroneous versions of the true variables we wish to capture. For instance, in EEOS, school quality is measured by variables like teachers’ years of experience that are much coarser (and different) than what a parent observes when she interacts with her child’s teacher, principal, and school. The point is that the regression will award the explanatory power to whichever measures best capture the variation in the true variables. The regression does not care whether the measure is labeled “family” or “school.” If a family measure captures school quality better than the school measures, the regression assigns the schools’ explanatory power to the family. I suggest that, as imperfect as family measures are, they may be more correlated with both the true family measures and the true school qualities than are the crude school measures.
My teacher example was deliberate. Coleman concluded that teachers did not matter because their characteristics (including years of experience in teaching; localism of teacher; teacher’s own education; and vocabulary score) explained only a small amount of the variation in achievement. This false analysis was repeated again and again by later scholars who ran Coleman-like regressions. This finding puzzled families because they believed that some teachers were much better than others. Today, we know that the families were right and Coleman was wrong. Numerous rigorous analyses of value-added demonstrate that teachers matter a great deal. How could Coleman have misled so many scholars? He failed to see that “good” families might be those who could discern which teachers were effective and get their children into those teachers’ classes. Thus, part of the apparent family effect was really a choose-effective-teachers effect. Indeed, since parents have opportunities to observe teachers directly, no one should be surprised if parents’ characteristics are more correlated with teachers’ value-added than are coarse background measures. But because Coleman did not think about selection, he thought that he had rigorously tested teachers’ effects. He was wrong, and his specious conclusions may have misdirected policy for decades. Only very recently have policymakers begun to focus on identifying and retaining teachers with high value-added.
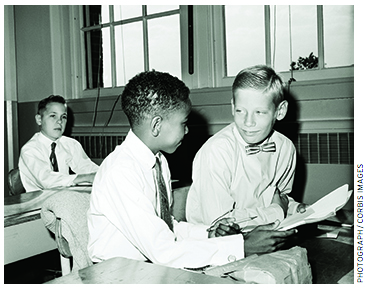
Similarly, Coleman’s conclusion that schools did not matter may have forestalled wise policymaking for decades. Rigorous lottery-based evaluations now consistently suggest that charter schools with no-excuses philosophies can greatly raise the achievement of disadvantaged urban students. Yet the vast majority of these students still do not have the opportunity to choose such schools, and Coleman is part of the reason they do not. Because he did not consider the possibility that advantaged children might have had high achievement precisely because their parents could choose good schools and ditch bad schools, policymakers felt comfortable denying school choice to disadvantaged families for decades. Perhaps if he had taken selection seriously, school choice experiments might have taken place much earlier.
Yet another example of the problems caused by Coleman’s lack of differentiation between causality and correlation is another of the most-cited EEOS takeaways: minority children were better-off in desegregated schools. Coleman’s interpretation was that this phenomenon occurred through peer effects: black students’ achievement improved when they had white classmates. This second conclusion had an enormous influence on policy, spawned any number of subsequent studies, and was the topic of much of Coleman’s own post-EEOS research. But nothing in the EEOS warranted the conclusion in the first place. The study did not rely on policy experiments such as students being quasi-randomly assigned. Instead, it relied on existing school environments that were the result of individual household choices. While it is possible that the black children in integrated schools had higher achievement because their white classmates causally affected them, it is just as possible that the sort of black families who were motivated and able to live in integrated neighborhoods were advantaged in numerous hard-to-observe ways. This is especially significant because the EEOS data predate nearly all desegregation orders that were sufficiently mandatory to have generated any quasi-random variation. Instead, the EEOS reflects an era when desegregation took voluntary forms. Looking back, it is obvious that this early and voluntary desegregation was dominated by selection, that is, families’ own choices. It is not that schools desegregated by literally hand selecting black families, but the mechanisms in place favored black families who were unusually prepared to live with whites. Often, the blacks were professionals who already spent most of their working lives among whites, had white friends, and participated in mixed-race church and social groups. At least that was the case in Shaker Heights, Ohio, the community in which I attended a voluntarily integrated school district.
Further, my own research, documented in the paper mentioned above, demonstrates that, when students are randomly assigned to schools, it is the achievement and not the race of their peers that matters. In other words, evidence based on more scientific methods suggests that Coleman’s conclusions were misleading.
Putting Coleman in the Past
I have provided examples in which Coleman’s specious methods caused decades of confusion and policy misdirection, but I would derogate his methods even if they had happened to produce findings that were consistently confirmed by better methods. Getting it right by chance is not a justification for methods that can mislead. Indeed, I now look back with some concern on a piece I wrote in 2001 titled, “If Families Matter Most, Where Do Schools Come In?” In it, I demonstrated that one could use modern data and easily reproduce EEOS-type correlations that, if interpreted naively, suggest that families matter and that schools and neighborhoods do not. I have deliberately abstained from such a demonstration in this article because that earlier demonstration apparently obscured the whole point of that piece. (Approximately 99 percent of readers misunderstand it.) The piece was intended to demonstrate that 1) good outcomes are associated with good choices made by families and thus 2) we cannot conclude that schools and neighborhoods do not matter because such conclusions are invalidated by selection; that 3) we cannot tell whether “bad” families are inefficacious because they only have bad choices open to them or because they would make bad choices even if offered good ones; and 4) we ought to be far more open to any policy that makes better choices available to families who now have little or no choice open to them.
I hope that I have now made these points clearly. I blame myself for making them too gently before: as a young scholar, I was averse to censuring Coleman’s faulty reasoning. This time, however, I want to incur no blame if scholars continue to be confused about the conclusions (or lack thereof) that we can derive from the EEOS. It is time that we, respectfully, ring down the curtain on the EEOS. Henceforth, let us dedicate all our efforts to analyses of families, schools, neighborhoods, and peers that employ credibly causal methods.
Caroline M. Hoxby is professor of economics at Stanford University.
This article appeared in the Spring 2016 issue of Education Next. Suggested citation format:
Hoxby, C.M. (2016). The Immensity of the Coleman Data Project: Gaining clarity on the report’s flaws will improve future research. Education Next, 16(2), 64-69.