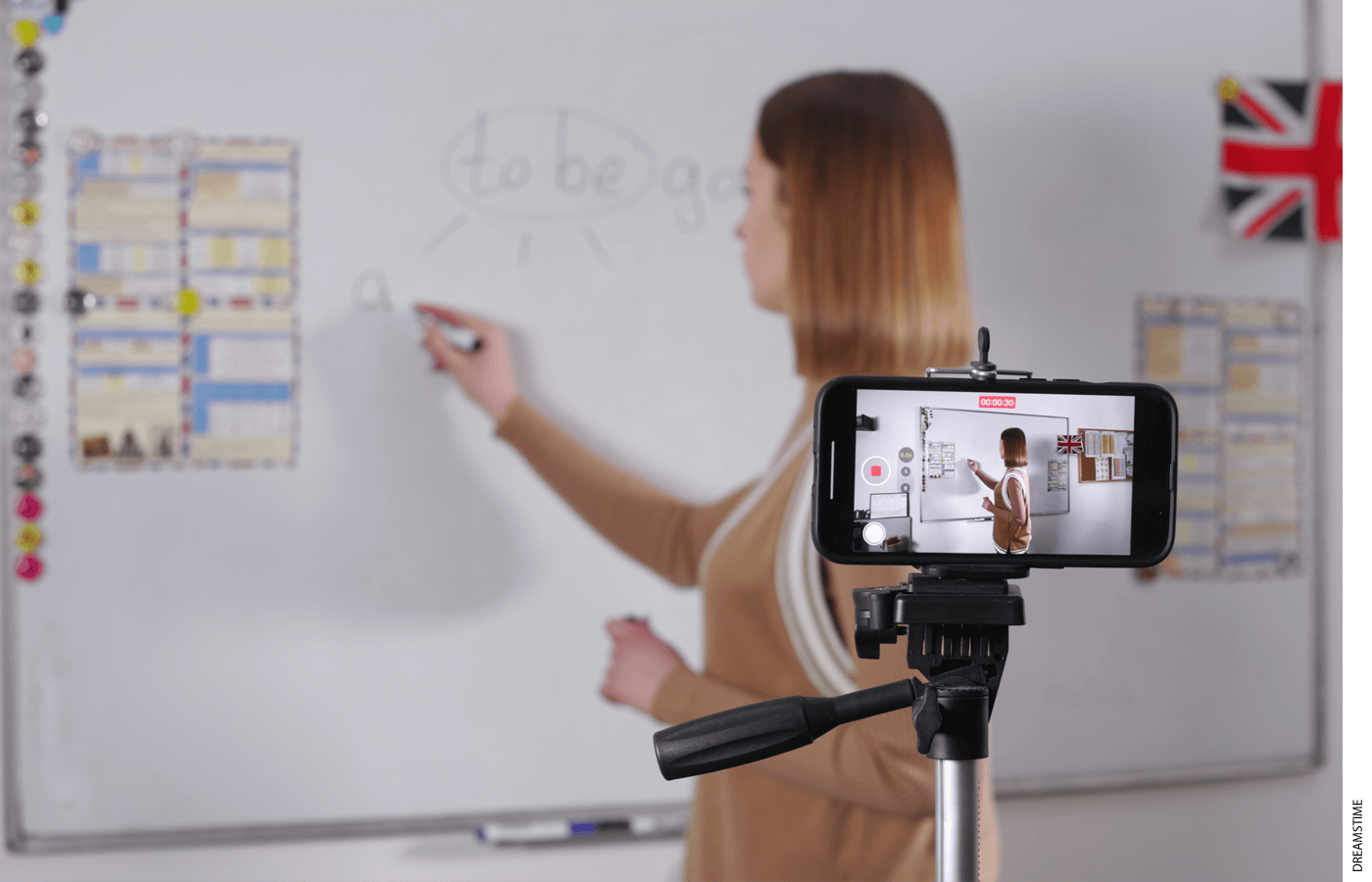
As is typical for edtech hype, the initial burst of enthusiasm for artificial intelligence in education focused on student-facing applications. Products like IXL, Zearn, and Khan Academy’s chatbot Khanmigo could take on the heavy lifting and personalize instruction for every kid! Who needs tutors, or even teachers, when kids can learn from machines?
Thankfully, the real-life limits of AI instruction surfaced quickly, given how hard it is for non-humanoids to motivate children and teens to pay attention and persist through hard work for any length of time (for example, see “The 5 Percent Problem,” features, Fall 2024). The apps are still popular, but it’s not clear that AI will crowd out live human instruction anytime soon.
If AI can’t replace teachers, maybe it can help them get better at their jobs. Multiple companies are pairing AI with inexpensive, ubiquitous video technology to provide feedback to educators through asynchronous, offsite observation. It’s an appealing idea, especially given the promise and popularity of instructional coaching, as well as the challenge of scaling it effectively (see “Taking Teacher Coaching To Scale,” research, Fall 2018).
While these efforts seem tailor-made for teachers looking to improve, there are clear applications across the spectrum of effectiveness. Like bodycams worn by police, video recordings and attendant AI tools could open a window into every classroom, exposing poor performers to scrutiny and helping to keep bad behavior in check.
Apps for Observations
Video-based observations are not new. The underlying, pre-AI idea is for teachers to record themselves providing instruction, choose some of their best samples, and upload those clips to a platform where an instructional coach or principal can watch and provide feedback. Indeed, this model was an important innovation of the Measures of Effective Teaching (MET) project launched in 2009 by the Bill & Melinda Gates Foundation (see “Lights, Camera, Action!” What Next, Spring 2011).
Edthena is one company that has built out a coaching-via-video-feedback service. Its founder, Adam Geller, started as a science teacher in St. Louis before moving on to the national strategy team at Teach For America. At the time, the organization was looking for a way to provide more frequent feedback to its corps members, given growing evidence that the best professional learning comes from educators regularly reviewing, discussing, and critiquing instructional practice together. It’s hard for instructional coaches or principals to visit every teacher’s classroom with much frequency, but recorded lessons allow anyone to observe and deliver feedback anytime from anywhere. That gave Geller an idea, which he later turned into Edthena.
For more than a decade, Geller claims, his platform has narrowed the “feedback gap” dramatically. Research studies find that video coaching via Edthena can improve teacher retention, competence, and confidence. Still, it is a large investment in staff resources. After all, coaches or administrators must find time to watch the videos and offer feedback, and there are only so many hours in the day.
Enter AI. Edthena is now offering an “AI Coach” chatbot that offers teachers specific prompts as they privately watch recordings of their lessons. The chatbot is designed to help teachers view their practice relative to common professional goals and to develop action plans to improve.
To be sure, an AI coach is no replacement for human coaching. An analogy might be the growing number of mental health chatbots on the market, many of them based on cognitive behavioral therapy (CBT), which can help patients reflect on their own thoughts and feelings and help them see things in a more constructive way. In the same way, Edthena’s AI Coach is helping teachers engage in “deep reflection about the classroom teaching,” Geller says. And because the AI tool is responding to teachers’ own self-evaluations, and not the lessons themselves, it’s relatively straightforward to train.
Gathering Data for Self-Improvement
If Edthena is about “deep reflection,” then TeachFX is about hardcore data. The app captures audio recordings from the classroom and uses voice recognition AI to differentiate between teacher and student speech during lessons. Teachers receive visualizations of class time spent on teacher talk, student talk, group talk, and wait time to assess student engagement, as well as more sophisticated analyses of verbal exchanges during class. It’s like a Fitbit for instruction.
TeachFX founder Jamie Poskin, a former high school teacher, got the idea while interviewing a school principal as a Stanford University graduate student. They discussed the challenge of providing feedback to teachers, especially new ones. Recording lessons was intriguing, they agreed, but when could principals find the time to watch the videos? The principal wondered, what if AI could be trained to look for the indicators of good practice—the teacher “moves” that are universally applicable regardless of grade level or subject matter?
The first version of TeachFX focused on a single metric: teacher talk versus student talk, based on voluminous research evidence that the more kids talk during direct instruction, the more they tend to learn. And though classrooms can be cacophonous (especially elementary ones), the technology could readily distinguish between teacher and student voices. Not only were such analyses doable, according to internal company data, but also just turning on the TeachFX app helped teachers more than double the amount of student talk during class. According to the company, almost 80 percent of teachers in a typical implementation use the tool on a recurring basis.
Over time, as the technology has improved, the platform added more metrics aligned with evidence-based best practices. For example: What proportion of a teacher’s questions are open-ended? How long is she waiting for students to answer? A study by Dorottya Demszky and colleagues published in 2023 found that teachers receiving feedback from TeachFX increased their use of “focusing questions,” which prompt students to reflect on and explain their thinking, by 20 percent.
A Role for AI in Evaluation?
It’s one thing to use AI to provide constructive, no-stakes feedback to teachers about their instructional practice. But what about incorporating it into formal performance evaluations?
Nobody I talked to liked that idea.
Thomas Kane of the Harvard Graduate School of Education, who ran the MET project, said, “AI could make it easier for teachers to get more frequent feedback, without the taint of a supervisory relationship.” But introduce that “supervisory relationship,” and you lose teachers’ willingness to give these technologies a try.
Indeed, neither company founder I spoke with was eager to see their tech used for teacher evaluations. As TeachFX’s Poskin told me, “You want teachers to learn and grow.” The more often teachers upload recordings to the platform, the better. Yet formal evaluations usually only happen every few years. They are the antithesis of constructive feedback.
That said, leaders of both companies welcome teachers’ deciding to use their recordings, or the data and “reflection logs” derived from them, in coaching sessions or formal evaluations. In all cases, the key is leaving those decisions to teachers and letting them keep control of the process and data.
To me, these apps sound like great tools for conscientious teachers eager to improve—as Geller and Poskin no doubt were. But it strikes me that teacher motivation to use them as intended must be an issue, just as it is for students. Teachers are crazy-busy, and apps like these are, ultimately, extra work.
To their credit, some districts provide incentives, such as counting the time teachers spend using the apps against professional learning requirements or allowing recordings to stand in for weekly classroom walkthroughs. Those are steps in the right direction—but we shouldn’t expect uptake to be universal. To me, it seems likely that the worst teachers, who arguably would have the most to gain, are the least likely to engage with these sorts of technologies.
From Bodycams to Classroom Cams
I don’t think it would be crazy, then, for someone to develop a version of this idea that is less about helping well-meaning teachers get better, and more about holding the small number of ineffective teachers accountable. Our schools have long faced the “street-level bureaucrat” problem, coined by political scientist Michael Lipsky in 1969. The idea is that some government services depend so much on the judgment and discretion of people on the ground that it’s hard to evaluate their work or hold them accountable. Teaching is one of those fields; policing is another.
In the world of law enforcement, dash cams and bodycams have changed the equation by providing a clear record of police officers’ interactions with the public, for good or ill. No doubt this has spurred all manner of questions and challenges, such as when to release footage, how to interpret it, and what is admissible in court. Bodycam mandates have garnered some support along with serious concerns about privacy and reliability. But there’s little doubt that police brutality and misconduct face greater scrutiny now than in the past.
So why not bring the same line of thinking into public schools? Put cameras and microphones in every classroom. Turn them on and keep them on. Send the recordings to the cloud and let machine learning do its thing (with strict privacy and security protocols in place, of course). If AI already can differentiate between good and bad questions, surely it can tell principals or department chairs if a teacher starts instruction late and ends it early, or shows movies every Friday, or allows kids to roam the hallways, or makes no effort to stop them from cheating on tests. If such technology could stop the most egregious forms of bad teaching, it might provide a significant boost to student achievement.
Alas, given education politics, that will probably remain just one wonk’s dream. In the meantime, let’s use AI to help as many motivated teachers as possible go from good to great.
Michael J. Petrilli is president of the Thomas B. Fordham Institute, visiting fellow at Stanford University’s Hoover Institution, and an executive editor of Education Next.
This article appeared in the Fall 2024 issue of Education Next. Suggested citation format:
Petrilli, M.J. (2024). Next-Gen Classroom Observations, Powered by AI: Let’s go to the videotape to improve instruction and classroom practice. Education Next, 24(4), 80-82.