In Salman Khan’s new book, Brave New Words: How AI Will Revolutionize Education (and Why That’s a Good Thing) (Viking, 2024), the Khan Academy founder predicts that AI will transform education by providing every student with a virtual personalized tutor at an affordable cost. Is Khan right? Is radically improved achievement for all students within reach at last? If so, what sorts of changes should we expect to see, and when? If not, what will hold back the AI revolution that Khan foresees? John Bailey, a visiting fellow at the American Enterprise Institute, endorses Khan’s vision and explains the profound impact that AI technology is already making in education. John Warner, a columnist for the Chicago Tribune and former editor for McSweeney’s Internet Tendency, makes the case that all the hype about AI tutoring is, as Macbeth quips, full of sound and fury, signifying nothing.
A World of Possibilities
by John Bailey
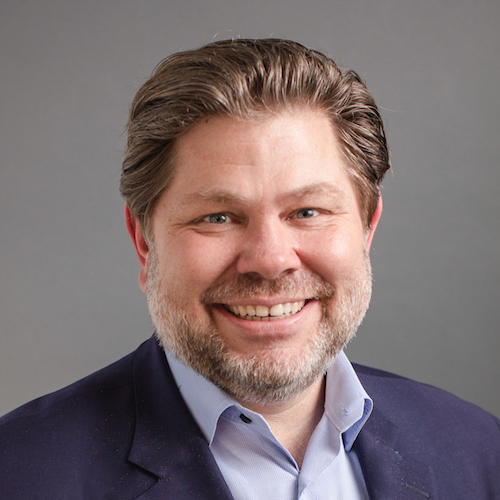
In his thought-provoking book, Brave New Words, Sal Khan discusses his early experimentation with generative AI, or GenAI, models and how, over time, they might change education. If AI is a new frontier, Brave New Words reads much like the field notes of an explorer documenting his experiences and trying to make sense of what they mean for teaching and learning.
At the heart of Khan’s vision is the idea of AI-powered tutors that adapt to each student’s unique needs, abilities, and interests. These advanced systems, he suggests, will provide direct instruction, real-time feedback, and personalized support, enabling students to learn at their own pace and master concepts more thoroughly than in traditional classroom settings.
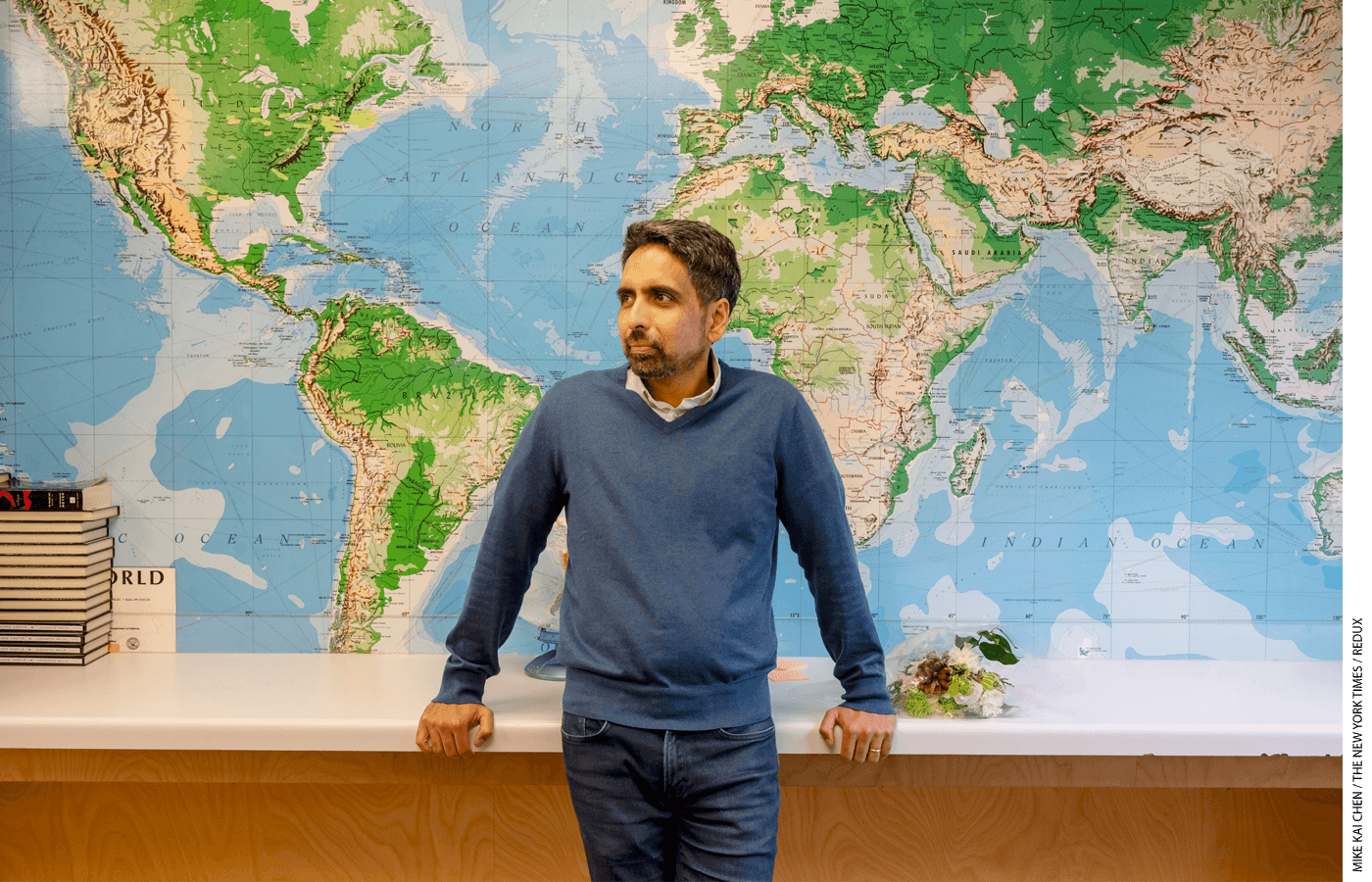
Khan discusses the early lessons his team is learning through Khanmigo, an AI-powered tutoring platform built around OpenAI’s flagship GenAI model GPT-4. Rather than just giving answers, this platform supports students by breaking down complex problems into manageable steps and providing explanations that guide students toward deeper understanding. Khanmigo can also assist students with their writing tasks, offering feedback and suggestions to improve their essays and helping them develop critical thinking skills.
In some respects, this vision is not entirely new. Educators have heard predictions about personalized learning solutions for decades, only to be disappointed by technologies that overpromised and underdelivered. It’s reminiscent of the classic Peanuts comic-strip scenario where Lucy time and again pulls away the football at the last second, causing Charlie Brown to fall flat on his back. Educators have seen wave after wave of hyped ed-tech solutions that sounded great in theory but fell short in practice. Many will feel a sense of déjà vu when they hear Khan’s vision of AI-powered personalized tutors, wondering if this is just the latest in a long line of footballs destined to be yanked away, leaving them disillusioned and disappointed.
So what makes this moment different? Why should educators believe that AI-powered tutoring systems like those envisioned by Khan will succeed where previous attempts at tech-facilitated personalized learning have fallen short?
I’m persuaded by Khan’s enthusiasm in part because of my own experience working with GenAI models over the last year, including participating in some early access and safety testing programs for several of the leading AI companies. What has struck me is that GenAI represents a paradigm shift that goes beyond previous innovations like the printing press or the Internet. While these earlier breakthroughs democratized access to information, AI goes a crucial step further by providing access to expertise. Books and the Internet serve as vast repositories of human knowledge, expanding our collective information base. However, they still require human intelligence to process, interpret, and apply that information effectively. AI, in contrast, not only stores and retrieves information but also simulates human-like intelligence to analyze, synthesize, and generate insights from it. That gives people the ability to apply on-demand expertise to a wide range of problems or tasks, including those common in education such as analyzing data, creating instructional materials, offering pedagogical insights, or brainstorming ideas.
AI-Powered Tutoring
As Khan’s book illustrates, the capabilities of these GenAI models make them uniquely suited to serve as tutors that can reflect a variety of teaching strategies, such as adopting a Socratic approach to a lesson or helping students reflect on their work. These general capabilities of GenAI can be fine-tuned to interact with custom data sets such as research findings, the school’s curriculum, or past student assessments. This gives GenAI more specific expertise, which can help drive coherence and ensure a consistent and integrated learning experience that reflects the school’s instructional goals and is based on rigorous research.
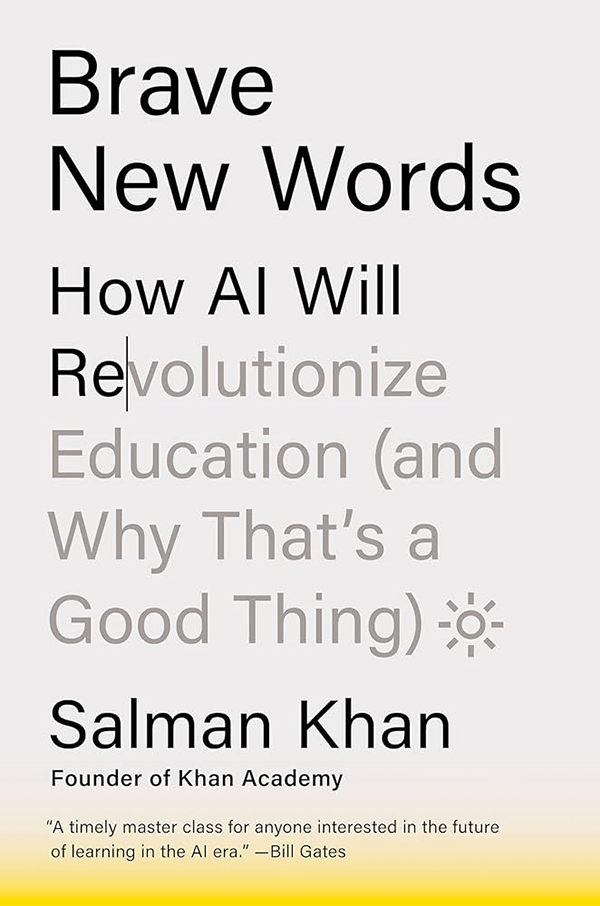
The high IQ of these models is now being matched by another, surprising characteristic—high EQ. GenAI produces fluent language that closely imitates the way humans talk and respond. In fact, a growing body of research shows not only that these models can provide accurate responses, but also that human evaluators rate their responses as more empathetic than those of other humans. Additional research is showing how this capacity might enable the GenAI system to better “understand” the emotional state of a user and respond appropriately. This can potentially allow an AI tutor to encourage, reassure, and motivate students and provide feedback to teachers on whether their lecture is engaging or boring.
Since the publication of Brave New Words, new capabilities have emerged among GenAI models that can enhance the tutoring experience. For example, many models now have the ability to analyze images, allowing students to upload a photo of a textbook page and receive instant assistance in understanding complex concepts.
Google’s Gemini 1.5 Pro goes one step further with the ability to analyze videos. An educator can provide it with a video of their instruction and ask questions about the video’s content just as easily as they could with a research paper. That capacity could provide a powerful tool to inform teacher practice or assess students. It also opens up an entirely new approach to training AI tutors based on analysis of videos that depict effective human tutors engaging with students.
Claude 3.5 Sonnet has introduced Artifacts, a feature that enables the AI model to generate small interactive resources alongside its text responses. In a physics course, Claude can generate simulations and interactive problem sets that allow students to apply their knowledge and see the implications of scientific principles.
OpenAI’s Advanced Voice technology has made significant strides in generating AI speech that closely mimics human speech patterns. The model incorporates subtle nuances such as simulated breathing sounds, filler words (like “um” and “uh”), laughter, and emotional inflections, to create a more natural and human-like listening experience. Additionally, the technology has the capability to detect and respond to users’ emotions, allowing for more empathetic and context-aware interactions.
This advancement should facilitate the development of AI tutors that are far more conversational and engaging than anything students have encountered with previous education technology. If a student is frustrated or confused, the AI model can adjust not only its response but also its tone, offering reassurance and encouragement. In fact, major AI companies are concerned that these systems could become too relational. OpenAI has cautioned that human-like voice interactions could lead users to anthropomorphize the AI tutor and develop an emotional connection or reliance on it. Early testing surfaced risks with extended interaction that could change social norms and cause some users to prefer engaging with the AI bot over human interaction, an issue that the Christensen Institute’s Julia Freeland Fisher has warned about.
These are all emerging capabilities that may still have some limitations, but they will continue to evolve and improve over time. The best way to think about these capabilities is as building blocks, like LEGO pieces, that can be assembled and configured to create innovative tools and services. What could only have been a text-based tutoring system a year ago can now engage students through active listening and conversation using speech recognition and synthesis. Student work, including visual elements, can be analyzed through advanced image analysis techniques. And empathetic capabilities can be adjusted to provide appropriate levels of encouragement or motivation to help guide a student through their lesson, adapting to their individual needs and progress.
Answering the Skeptics
These capabilities have generated much excitement, but this latest generation of AI has also been met with skepticism from some observers, who are wary of becoming distracted by yet another “silver bullet” technology fad. Critics point to the limitations of current models, worry that the hype could lead to diverting funds and attention away from critical education priorities, and argue that the focus should remain on addressing the complex, systemic issues that have long plagued the sector.
These are understandable and, to some extent, reasonable concerns. However, dismissing the potential impact of GenAI based on its current limitations is shortsighted, as the rapid pace of advancements suggests that these models will likely overcome many of their shortcomings in short order.
Skeptics whose only experience with GenAI is limited to the free version of ChatGPT 3.5 from 2023 may not fully grasp the advancements made in the field. In just one year, ChatGPT-4, once the leading frontier model, has been joined by a host of powerful contenders, including Google Gemini 1.5 Pro and Anthropic’s Claude 3.5 Sonnet, as well as new open models such as Meta’s Llama 3.1 and Mistral Large 2. Each of these models has unique strengths, excelling in different areas and tasks, and some do better with tutoring prompts than others. Just recently, OpenAI released a new model that is breaking AI records in complex reasoning, math, and science. These models will continue to get cheaper, faster, and more powerful over time, which will help support more experimentation in tutoring applications.
In some cases, disappointing GenAI output may be the result of the poor prompts it receives rather than the limitations of the technology. A growing body of research is showing that well-crafted prompts can dramatically improve an AI system’s performance on various tasks. Techniques such as assigning the model a particular role or expertise, providing relevant examples, guiding the AI’s reasoning process, or breaking complex problems into smaller steps can lead to much better results.
Critics are quick to point out errors or low-quality GenAI outputs, but quality control is also a challenge with traditional approaches. A RAND study found that educators often use platforms like Pinterest and Teachers Pay Teachers for instructional ideas, even though these sources tend to be of low quality and can contain their own “hallucinations” and biased content. Just over a third of teachers use at least one standards-aligned ELA curriculum, and less than half regularly use at least one aligned mathematics curriculum. Interventions like high-dosage (human) tutoring, which showed significant gains in smaller studies, have struggled to provide the same results when scaled, and survey research suggests these interventions are not reaching the students who need it the most. If the only contribution of a fine-tuned GenAI to the field of education is to accelerate the transition to high-quality instructional materials and support greater curricular coherence, it would still represent a worthwhile and arguably transformative advancement.
A nascent but growing body of research illustrates the effectiveness of GenAI tutors. For instance:
- Tutor CoPilot, a human-AI system that provides expert-like guidance to tutors, improved student mastery of topics by 4 percentage points in a randomized controlled trial with 1,800 students. Lower-rated tutors saw the greatest benefit, with their students improving mastery by 9 percentage points. Tutor CoPilot helped less-effective tutors achieve outcomes comparable to more-effective peers.
- A Harvard study found that students using a custom-designed AI chatbot tutor for a physics course showed approximately double the learning gains and significantly higher engagement compared to those in a traditional classroom. The AI tutor’s personalized feedback and students’ ability to self-pace proved especially beneficial when students were encountering new material.
- In Ghana, an AI-powered math tutor called Rori, accessible via WhatsApp, led to significantly higher math growth scores for students who used it for one hour per week, with an effect size equivalent to an extra year of learning. Rori’s low cost of $5 per student suggests it could be a cost-effective intervention in educational settings with limited resources.
- One study introduced Bridge, a method that employs a task analysis to model the decisionmaking processes that expert teachers use when they address student math errors. Researchers applied this method to a data set of 700 annotated real-world tutoring conversations with students from Title I schools. They found that when GPT-4 was given information about an expert teacher’s decisionmaking processes (including the type of mistake, teaching strategy, and response goal), the AI system’s responses to students’ math mistakes were rated 76 percent better by humans compared to when GPT-4 had to respond without that expert guidance. This study demonstrates the importance of incorporating expert knowledge into AI models for tutoring and other uses.
- In a randomized controlled study, students using an AI tutor demonstrated significantly greater learning gains in less time compared to those in an active learning classroom. The AI-using students spent a median of 49 minutes on the tasks compared to the 60-minute lecture. AI-using students reported higher levels of engagement and motivation, with 83 percent considering the AI’s explanations as good as or better than human instructors’. The AI tutor’s effectiveness was attributed to its meticulous adherence to pedagogical best practices, including active learning, cognitive load management, growth mindset, scaffolding, accuracy, timely feedback, and self-pacing.
- One study demonstrated how AI tutors can act as education experts, successfully replicating known teaching principles and creating improved math worksheets that significantly align with teacher judgments. Those capacities suggest that AI could speed up lesson design while highlighting the continued importance of human expertise and real student testing.
- A field experiment with nearly 1,000 students in a Turkish high school used GPT-4 during three tutoring sessions covering 15 percent of the curriculum. Researchers found that access to the AI tutors significantly improved math performance (by 48 percent to 127 percent) but subsequently harmed educational outcomes when access was removed (17 percent reduction), suggesting students used GPT-4 as a “crutch” instead of actually learning critical skills. However, safeguards in the GPT tutor largely mitigated these negative effects, highlighting the need for caution when deploying generative AI to ensure long-term productivity through continued human learning.
The AI-powered tutoring systems of the near future are likely to be significantly more capable than today’s. When critics talk about GenAI not fully understanding a student or failing to build on the student’s previous learning, they’re ignoring how fast these models are becoming more relational. AI tutors will gain access to larger memory and context windows, including the ability to read and analyze a student’s previous work to better inform tutoring sessions. They will soon be able to see and listen to students, opening up new ways of engaging them and assessing their understanding of concepts. There’s reason to believe that future versions of these systems will have even greater empathic capabilities, allowing them to better motivate and engage students.
That said, while GenAI is a powerful tool, it is just that—a tool. The value comes not from the tool itself but from how and when it is used. Educators should implement AI tutors in targeted ways to solve specific instructional challenges, not simply adopt them for their own sake. These tools should serve to support and empower educators, not replace them. Most important, the use of GenAI must be balanced against the need to cultivate students’ ability to focus and sustain attention—skills that today’s digital distractions increasingly threaten.
Moment of Urgency
These GenAI tools and capabilities are emerging at the exact moment when the education sector urgently needs innovative solutions. Chronic absenteeism surged to include 28 percent of all K–12 students in 2022, with only a slight improvement in 2023. A Walton Family Foundation–Gallup “Voices of Gen Z” study found that between 25 percent and 54 percent of Gen Z K–12 students report that they lack engaging experiences in school. The average student has regained only a fraction of the learning lost during the pandemic, with just one-third of math losses and one-quarter of reading losses recovered. According to research by the Northwest Evaluation Association, students will need an average of four additional months of learning to catch up, and in some cases, as much as nine.
Perhaps more traditional reforms and tutoring will be able to address these challenges. I certainly hope they will help, but I doubt that they’ll prove sufficient for the depth and breadth of the challenges we’re facing. The urgency of the moment should be a call to experiment and pilot new approaches that explore how best to thoughtfully and purposefully harness the capabilities of GenAI. We need more, not less, experimentation with AI tutors. We need more efforts using GenAI to lighten the administrative load that often distracts teachers from their most important work: building the deep, meaningful relationships with students that are the foundation of academic success.
The vision Khan presents in Brave New Words is not a distant dream but an unfolding reality that demands our attention and active engagement. The rapid advancements in GenAI have opened up a world of possibilities for improving teaching and learning, but we must approach this new frontier with both excitement and caution. Realizing the full potential of AI in education will require more than just technological innovation; it will demand a collective commitment to ensuring that these powerful tools are harnessed in ways that genuinely benefit all students. Khan’s roadmap may not be fully realized in the immediate future, but it sets a course for a destination worth pursuing—a world in which every student, regardless of background, has access to the personalized support, engaging learning experiences, and high-quality education they need to thrive. The future of our students, and our society, depends on our willingness to act decisively and creatively at this crucial juncture.
John Bailey is a non-resident senior fellow at the American Enterprise Institute.
A Case for Skepticism
by John Warner
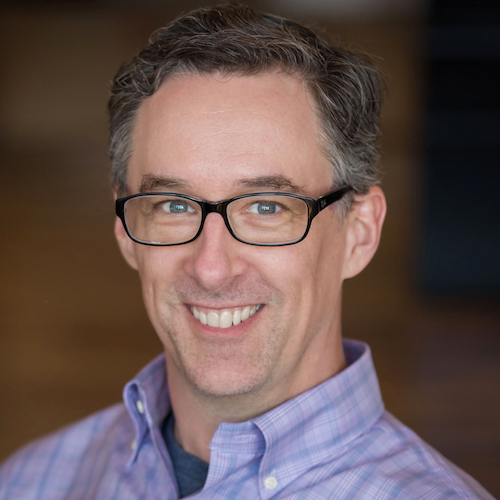
I have been called upon to provide the skeptic’s take on the proposition at hand, and I am happy to do so because I am indeed quite skeptical that generative-AI-driven tutor bots like Khanmigo will revolutionize education.
But I do not want to be only skeptical.
I also don’t want to give away my own ending, but long before generative AI arrived on the scene, I believed we’d taken a wrong turn in education—a case outlined in my book, Why They Can’t Write: Killing the Five-Paragraph Essay and Other Necessities. Teaching machines like Khanmigo, the ChatGPT tutor bot featured in Salman Khan’s Brave New Words, threaten to more deeply entrench the anti-learning practices that have been at the heart of education reform for the last 30 years.
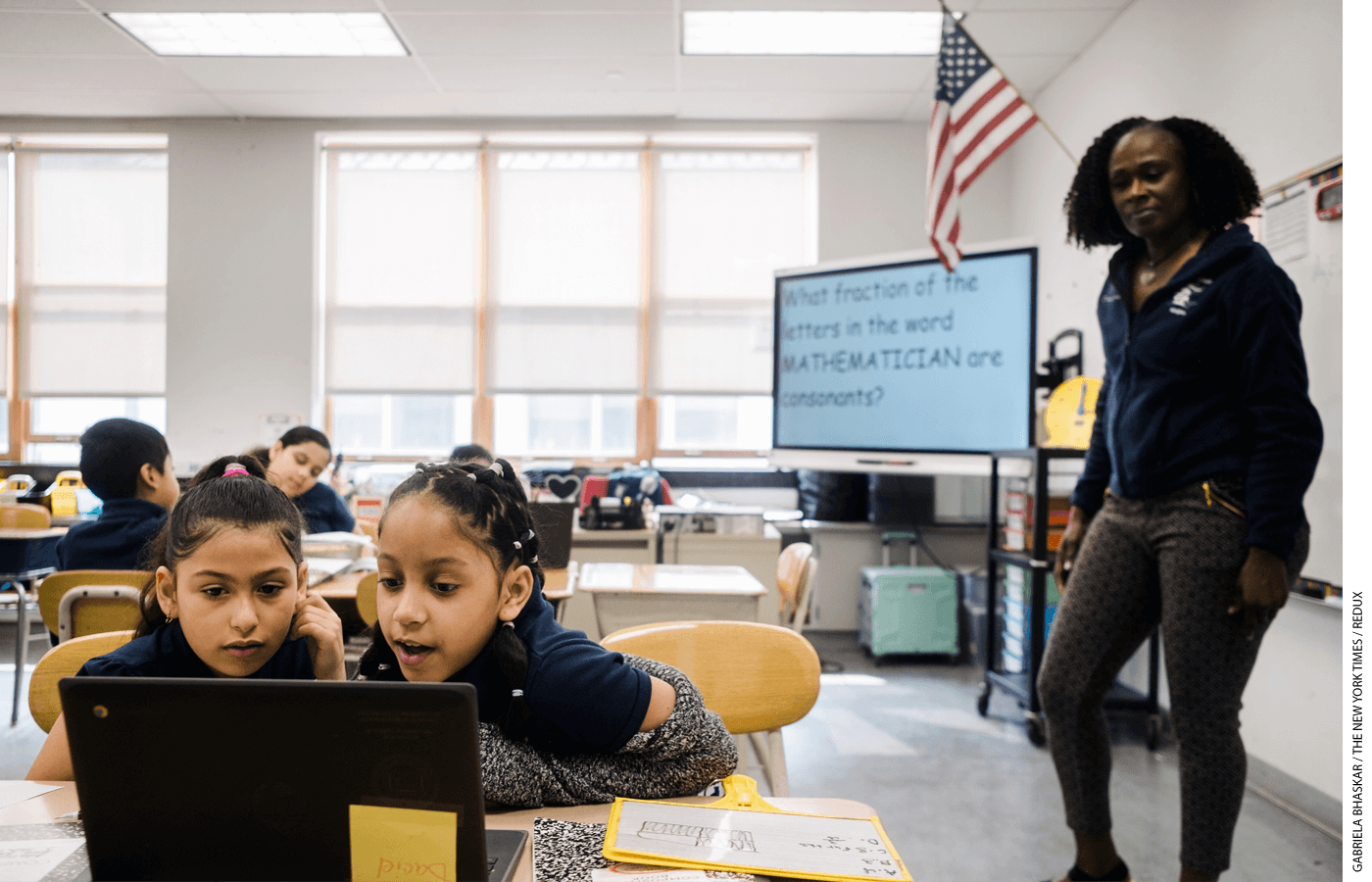
The people who have guided those failed efforts include some of the prominent endorsers of Brave New Words: Bill Gates, Laurene Powell Jobs, and former secretary of education Arne Duncan. I do not dispute the sincerity of their desire to improve education outcomes for students, but I do question their success and must wonder why we continue to put so much stock in their opinions.
Ultimately, I’m going to suggest that the quest to revolutionize education is the very thing that has led to students becoming increasingly disengaged, stressed, and anxious about school, without improving outcomes on any measure you care to name.
But first, let me air my case for skepticism, which primarily rests on the fact that when it comes to inventing the teaching machine, of which Khanmigo is the latest example, many before have tried and failed.
In the 1920s and ’30s, Sidney Pressey unsuccessfully pursued his vision of an “Automatic Teacher,” which was really a testing machine, designed to reward children with candy for correct answers. B. F. Skinner, the godfather of behaviorism, picked up the baton in the 1950s, certain that his work with training pigeons could be translated to teaching children.
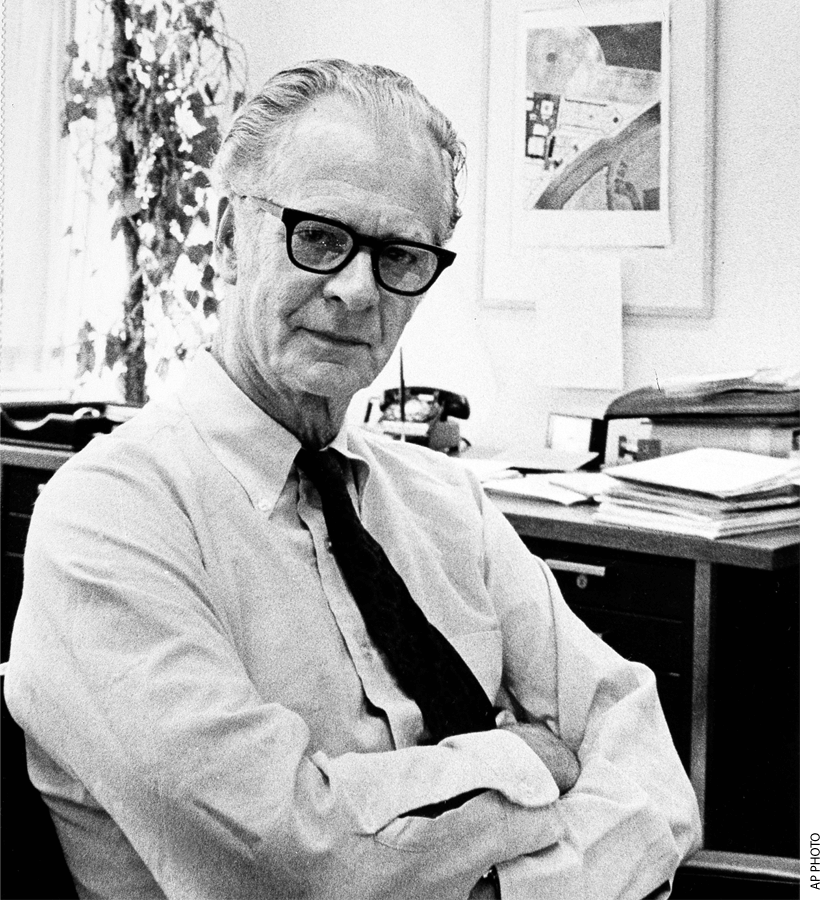
I am drawing from Audrey Watters’s indispensable history of education technology, Teaching Machines, in which she shows how the dreams of visionary men (and they have all been men) have been repeatedly dashed on the shoals of the complexity of learning and the sheer variety of human beings. We are not pigeons.
More recently we have had Knewton (the “mind-reading robo tutor in the sky”) and Amplify, which flushed $1 billion of Rupert Murdoch’s cash down the drain before pivoting to a new life as an instructional supplement to be used primarily in whole-class situations. IBM spent five years trying to build a personalized learning interface on their Watson platform before abandoning it in 2017 as a hopeless pursuit.
Conceptually, Khan’s vision is identical to his forebears. His goal is to provide an “artificially intelligent but amazing personalized tutor.” The only difference between previous teaching machines and Khanmigo is ChatGPT’s ability to generate responsive syntax to student inputs. Those who are believers in the power of generative AI will argue that these are sufficient to lift Khanmigo (and its ilk) above past attempts.
I am skeptical because, like all other attempts at personalized learning, Khanmigo relies on an algorithmic model of learning, which works like this:
1. Decide what students need to learn and sketch out the relationship between the different concepts and skills we believe are important. Call this a map.
2. Do some kind of diagnostics that allow us to place students on the map, where everything behind them is what they know, and everything in front of them is what they should learn.
3. Expose students to “learning objects,” using the algorithm to put the appropriate object in front of the student at the appropriate time.
4. Make the student use the learning object.
5. Measure what the student knows based on this interaction.
6. Resituate the student on the map, rinse, and repeat.
This approach to teaching and learning may begin to break down in a number of different places.
The first is that students are not so much on a map when working within this model, but on a line, a continuum, where they are expected to learn and generally move along a prescribed route. But learning does not happen on a continuum. At a given time, students may zoom off in any number of directions. Not forward or backward, but upward, downward, sideways, slantways, and any other ways you can think of! Students may (mentally) spin in circles or they may make a leap you had no reason to anticipate. To represent where a student might move on their learning journey, we do not need a line or a map but a sphere with infinitely expanding boundaries and an infinite number of different points that could be occupied.
Learning is (at least) a three-dimensional problem, not a two-dimensional one.
The Human Element
Another disconnect between what a tutor bot can offer and what happens in a human exchange between student and teacher is that learning is not just about what someone knows, but how someone is thinking. A wrong answer can have many different points of origin, and diagnosing the issue is a matter of using one’s judgment, a behavior that large language models are incapable of, but which is something human teachers do hundreds or even thousands of times a day.
For example, during my 20-plus years of teaching, I would often ask a student, “Does that make sense?” The times when their mouths said “yes” but their faces said “no” are beyond counting. In those cases, I had to continue to exercise my judgment to keep the students learning.
The biggest hurdle, however, is point number four: make the student use the learning object.
Sataya Nitta, who headed up the IBM Watson tutoring project, explained why the team was destined to fail, “We missed something important. At the heart of education, at the heart of any learning, is engagement.”
Writing in Education Next, Laurence Holt described what he dubbed “the 5 percent problem.” Holt observed that in many instances, online math programs—modern teaching machines—have demonstrated large positive effect sizes among research subjects. Khan Academy’s math-practice website, for example, was shown to contribute the equivalent of “several months of additional schooling” for “students who used the program as recommended.”
Despite the widespread adoption and apparent efficacy of these programs, overall student achievement has not improved. Why not?
Only 5 percent of students are using these programs as recommended. As Holt put it, “Imagine a doctor prescribing a sophisticated new drug to 100 patients and finding 95 of them didn’t take it as prescribed. That is the situation with many online math interventions in K–12 education today. They are a solution for the 5 percent. The other 95 percent see minimal gains, if any.” The overwhelming majority of students opt out of using the software. The 5 percent who do use it correctly are the small proportion of students who will seemingly do anything their teachers task them with.
I suppose it is possible that Khanmigo has some secret formula for ensuring student engagement, but Khan offers no evidence of that in his book. Instead, he engages in speculation, as when he suggests how interesting it must be to have a Rembrandt van Rijn chatbot ask if you like to paint. The lack of evidence in the book is understandable, given that Khanmigo’s beta version was launched in March 2023 and Khan’s book was published in May 2024. With the time it takes to draft, revise, edit, copy edit, print, and distribute a book, there would have been no time to gather any real-world data on how students are using Khanmigo. Khan has made his prediction on speculation extending from understandable amazement at what large language models seem to be able to do, rather than real-world trials.
There is a yearning to find intelligence and the ability to reason in the outputs of large language models, but these are the byproduct of what Baldur Bjarnason calls “the intelligence illusion,” a natural impulse to assign agency to what is, in reality, an automatic syntax-generating machine. To the extent a large language model can “reason,” we know that its form of reasoning looks nothing like that of a human.
There are other conceptual-level problems that Khan does not seem to have considered. For example, the always available tutor bot is touted as being able to offer “real-time” feedback, but there is no evidence that “real-time” (as opposed to the more useful “timely”) feedback aids learning. Real-time feedback is a tool of efficiency, but in what world is learning necessarily efficient?
In fact, when it comes to teaching writing—my area of focus when I taught—real-time feedback would do significant harm. Writing is, by necessity, a slow process of thought and consideration over a piece’s communicative purpose within a rhetorical situation. A real-time writing aid coaching students to complete an assignment as efficiently as possible threatens to short-circuit the necessary friction that allows for the building of writing skills.
Sometimes in working with students I would engage in detailed back-and-forths about what they intended with their writing versus what I was experiencing as the reader. Other times, I would read a student piece and simply say, “Not soup yet,” meaning I knew (and the student knew) the piece was not done. Knowing what to say to a student to keep them learning grew out of the human relationships I developed and the knowledge of a student’s writing I gleaned over time––things chatbots cannot yet simulate, let alone do for real.
Khan also frames AI as an aid to automating so-called lower-level teacher tasks such as lesson planning and grading, but only someone who has not taught would call these lower-level tasks. Would an orchestra conductor outsource the making of the performance program to AI?
Similarly, at least when it comes to student writing, grading is an essential teacher task because it is the best way to assess the evidence not only of what students have learned, but also how they’ve learned it (or haven’t learned it). Teaching a writing course and outsourcing the grading is akin to the orchestra conductor hearing only the audience’s level of applause rather than listening to the performance itself, or a football coach knowing the score but not watching the game. It’s literally nonsensical.
While I am skeptical that we are about to undergo an AI-powered education revolution, I do believe that we should aggressively and widely experiment with all methods that may help students learn. Let a hundred, a thousand, a million flowers bloom.
But these experiments should be sensible and proportional. As of March 2024, Khanmigo was reportedly being used by 65,000 students. Microsoft has provided the resources to make Khanmigo free to all students, an investment that is hard to measure. But given what we know about the “cost of compute” for ChatGPT, we must be talking about many millions of dollars.
The belief that generative AI will be transformative requires setting aside what we know about how and why previous attempts at transformation have fizzled. It calls to mind the scene in the film This Is Spinal Tap when Christopher Guest’s Nigel Tufnel shows Marty Di Bergi (Rob Reiner) his “special” Marshall amp that has a maximum volume of 11 rather than the standard 10, and Di Bergi asks, “Why don’t you just make 10 louder and make 10 be the top number?” Tufnel ponders this for a beat or two before saying, “These go to 11.”
Layering generative AI onto a personalized learning model is like Tufnel saying, “These go to 11,” a declaration that this technology is different simply because it has a higher number.
The theory that these innovations will be transformative is built on wishful thinking. The evidence at this time is scant, with significant prior experience suggesting that this model is destined to crash into the reality of human behavior.
If we’re going to help students learn, we need to start with what makes us human rather than getting carried away by AI automation.
EdNext in your inbox
Sign up for the EdNext Weekly newsletter, and stay up to date with the Daily Digest, delivered straight to your inbox.
The Disengaged Student
The number-one barrier to student learning in school is lack of engagement. Engagement is the gateway to learning, and it is lacking.
Data prior to the pandemic from Gallup showed that we had an “engagement crisis” in schools, with fewer than 50 percent of students in grades 5–12 saying they were “engaged” with school. A full one-quarter of students reported being “actively disengaged.”
A 2024 survey from Gallup, sponsored by the Walton Family Foundation, found that only between 11 percent and 33 percent of students “strongly agree” that they have even one of eight engaging classroom experiences (for example, having supportive teachers and feeling motivated and challenged). Between 2023 and 2024, almost every school-engagement measure declined. Fewer than three in five students report that, in a given week, they have learned “something interesting.”
Disengagement increases with each additional year of a student’s schooling. The problem is particularly acute for students who do not intend to pursue post-secondary education.
Gallup asked students what got them excited about learning. The top responses were:
- The topic was something I wanted to learn more about. (60 percent)
- The teacher made it exciting and interesting. (60 percent)
- I was able to learn in a hands-on way, such as doing an experiment, simulation or demonstration. (46 percent)
Second from the bottom was:
- The lesson used technology to help me learn. (23 percent)
While this generation is said to be obsessed with screens, they don’t appear particularly enthusiastic about screen-based experiences in school. Engagement comes through helping students relate to the material, and then giving them something meaningful to do.
Gallup also asked students to think about the best middle or high school teacher they’d ever had and what made them the best teacher. The top answers were:
- They cared about you as a person. (73 percent)
- They made it easy to understand what they are teaching. (62 percent)
- They were someone you trusted. (58 percent)
As previous experiments in personalized learning have demonstrated, teaching is more than just putting educational activities in front of students. Teaching requires being simultaneously aware of and responsive to both the relational and cognitive goals of the learner. Students must feel as though they are cared about, and a teacher must know how to convey the material in a way that allows students to learn.
As I found when I taught writing, the way to achieve this complex balance is constantly shifting, assignment to assignment, student to student, semester to semester. Adjusting to those shifts is the constant work of teaching. It is wonderful, but obviously difficult work, made more difficult by the less than ideal circumstances under which many teachers labor.
In his book Someone Has to Fail: The Zero-Sum Game of Public Schooling, education historian David Labaree looks at the school reforms touted by people like Bill Gates or think tanks like the American Enterprise Institute and says, “Only one thing is certain about the map that reformers create in their effort to see schooling: it leaves out almost everything. The complex ecology of the classroom disappears into the simplified columns of summary statistics.”
Our experiences of the last 30-plus years should be more than sufficient to show that learning is not something that can be determined by algorithms and much of what is meaningful about learning cannot be quantified.
We have created a system where school is predicated on what I call “indefinite future rewards,” in which the experience of the present is unimportant and all that matters is the payoff (college, career, and so on) down the line. This ethos has primarily served to make students miserable and definitely hasn’t helped them learn.
According to research from the Harvard Graduate School of Education, the generation that has experienced this type of schooling (18- to 25-year-olds) have rates of anxiety and depression double that of today’s teens.
Their chief source of anxiety is “a lack of meaning, purpose, and direction.”
I have seen the best minds of several generations dulled by a grim march through proficiencies, bored, anxious, and stressed over the pursuit of closing the gap between a B+ and an A-. It should be a scandal that our schools have not done more to help students find a sense of purpose and direction.
Students are clearly yearning for human connection. Why are we so resistant to providing it to them? If a teacher has too many students to attend to their needs, why are we not investing millions and even billions into changing that equation, rather than outsourcing our humanity to an algorithm?
We should want more for students than even the most wondrous teaching machines could ever offer.
John Warner is the author of Why They Can’t Write: Killing the Five-Paragraph Essay and Other Necessities and the forthcoming More than Words: How to Think About Writing in the Age of AI. He is on the affiliate faculty of College of Charleston.
This article appeared in the Winter 2025 issue of Education Next. Suggested citation format:
Baily, J., and Warner, J. (2025). AI Tutors: Hype or Hope for Education? Education Next, 25(1), 62-71.