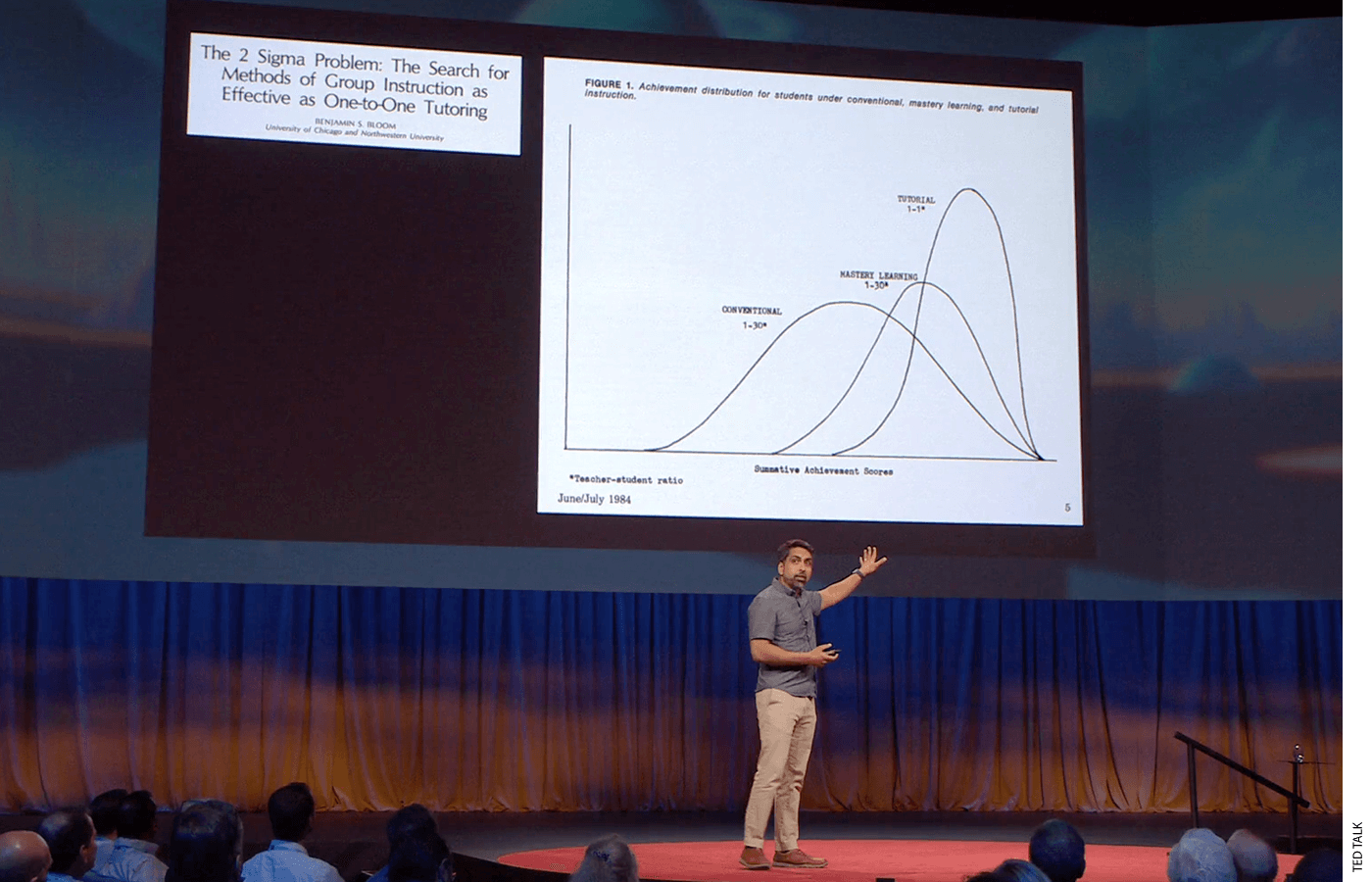
In the fall of 1945, when my father was not quite eight years old, his teacher told my grandmother that he was failing 2nd grade. My father doesn’t remember her reasons, or maybe my grandmother never told him, but the teacher felt he wasn’t ready for 2nd-grade work.
“If he’s not succeeding in 2nd grade,” my grandmother suggested, “why not try him in 3rd?” And she found a tutor, a retired teacher from a different school.
For seven weeks, my father met for an hour a day with the tutor, who gave him homework after each session. The tutor’s charge was to make sure my father mastered the curriculum, not just for 2nd grade but for enough of 3rd grade that he could slip into a 3rd-grade classroom in January 1946, a year early, without needing further help.
But the tutor overdid it. Not only did my father encounter nothing in 3rd grade she hadn’t taught him, but he coasted through 4th and 5th grade as well.
Around 1960, while shopping at Filene’s Basement in downtown Boston, my grandmother ran into an old neighbor—a mom who’d moved away when my grandmother was seeking a tutor to help her son escape from 2nd grade. After bragging about her own family, the neighbor asked if my father was all right.
“He’s fine!” said my grandmother triumphantly. “He’s at Oxford, on a Rhodes Scholarship.”
Stories like this give the impression that tutors can work miracles. For centuries after Aristotle tutored Alexander the Great, certain fortunate individuals—including Albert Einstein, Felix Mendelssohn, Agatha Christie, and practically every British monarch before Charles III—were educated partly or entirely by private tutors and family members. While no scholar regrets the spread of mass schooling, many suspect that the instruction students receive from a teacher in a large classroom can never match the personalized instruction that comes from a tutor focused only on their individual needs.
In a 1984 essay, Benjamin Bloom, an educational psychologist at the University of Chicago, asserted that tutoring offered “the best learning conditions we can devise.” Tutors, Bloom claimed, could raise student achievement by two full standard deviations—or, in statistical parlance, two “sigmas.” In Bloom’s view, this extraordinary effect proved that most students were capable of much greater learning than they typically achieved, but most of their potential went untapped because it was impractical to assign an individual tutor to every student. The major challenge facing education, Bloom argued, was to devise more economical interventions that could approach the benefits of tutoring.
Bloom’s article, “The 2 Sigma Problem,” quickly became a classic. Within two years of its publication, other scholars were citing it weekly—50 times a year—and it has only grown in influence over the decades. In the past 10 years, the article has been cited more than 2,000 times (see Figure 1).
The influence of Bloom’s two-sigma essay reached well beyond the scholarly literature. As the computing and telecommunication revolutions advanced, visionaries repeatedly highlighted the potential of technology to answer Bloom’s challenge. Starting in the 1980s, researchers and technologists developed and eventually brought to market “cognitive computer tutors,” which Albert Corbett at Carnegie Mellon University claimed in 2001 were “solving the two sigma problem.” In the 2010s, improvements in two-way video conferencing let students see human tutors at off hours and remote locations, bringing the dream of universal access closer—though there were still simply not enough tutors to go around.
Then, in late 2022, startling improvements in artificial intelligence offered students a way to converse with software in flexible, informal language, without requiring a human tutor on the other end of a phone or video connection. Sal Khan, founder of Khan Academy, highlighted this promise in a May 2023 TedX talk, “The Two Sigma Solution,” which promoted the launch of his AI-driven Khanmigo tutoring software.
Enthusiasm for tutoring has burgeoned since the Covid-19 pandemic. More than two years after schools reopened, average reading scores are still 0.1 standard deviations lower, and math scores are 0.2 standard deviations lower, on average, than they would be if schools had never closed. The persistence of pandemic learning loss can make it look like an insurmountable problem, yet the losses are just a fraction of the two-sigma effect that Bloom claimed tutoring could produce. Could just a little bit of tutoring catch kids up, or even help them get ahead?
Are Two-Sigma Effects Realistic?
But how realistic is it to expect any kind of tutoring—human or AI—to improve student achievement by two standard deviations?
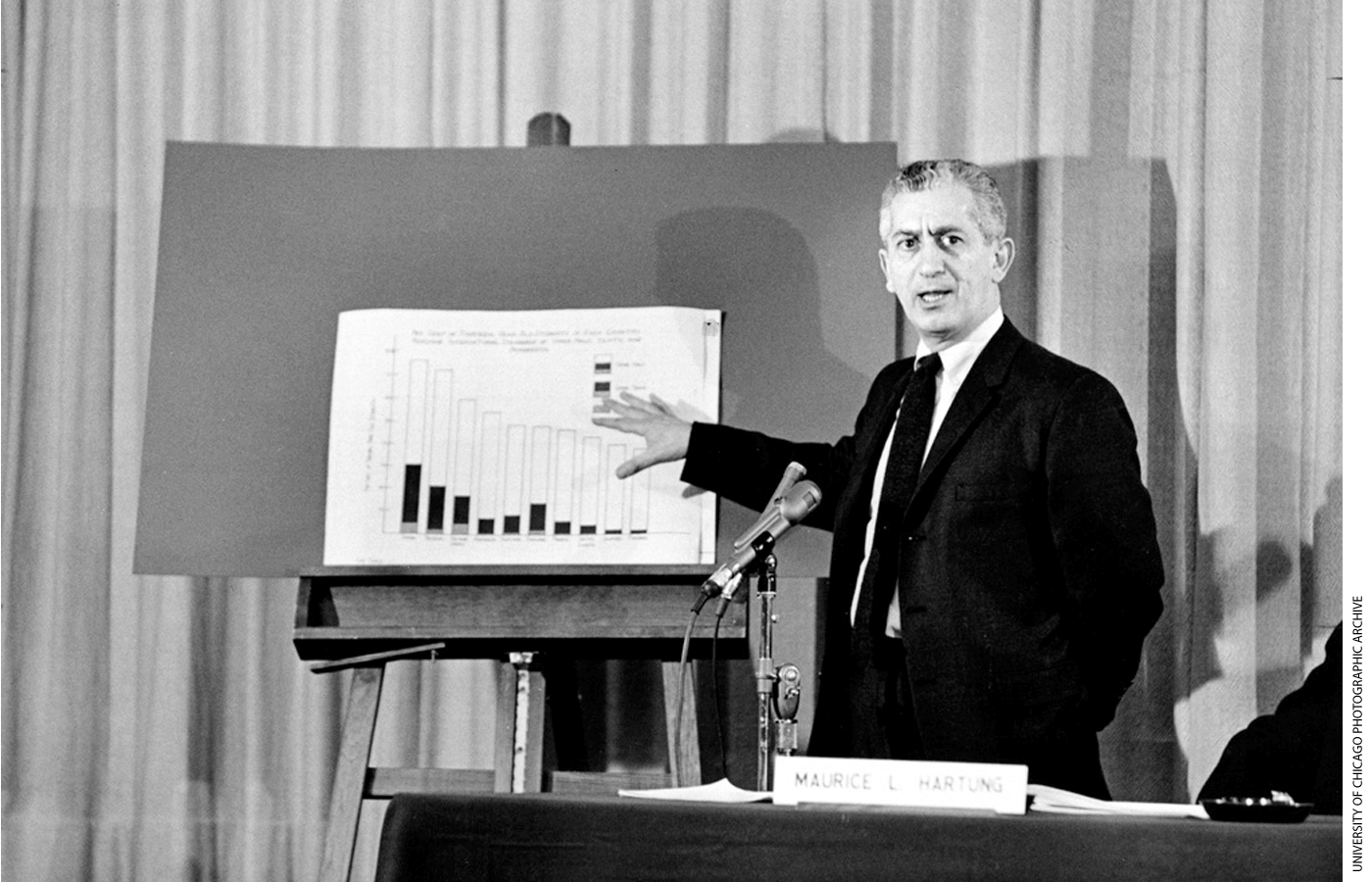
Two sigmas is an enormous effect size. As Bloom explained, a two-sigma improvement would take a student from the 50th to the 98th percentile of the achievement distribution. If a tutor could raise, say, SAT scores by that amount, they could turn an average student into a potential Rhodes Scholar.
Two sigmas is more than twice the average test score gap between children who are poor enough to get free school lunches and children who pay full price. If tutors could raise poor children’s test scores by that much, they could not only close the achievement gap but reverse it—taking poor children from lagging far behind their better-off peers to jumping far ahead.
Two sigmas also represents an enormous amount of learning, especially for older students. It represents more than a year’s learning in early elementary school—and something like five years’ learning in middle and high school.
It all sounds great, but if it also sounds a little farfetched to you, you’re not alone. In 2020, Matthew Kraft at Brown University suggested that Bloom’s claim “helped to anchor education researchers’ expectations for unrealistically large effect sizes.” Kraft’s review found that most educational interventions produce effects of 0.1 standard deviations or less. Tutoring can be much more effective than that but rarely approaches two standard deviations.
A 1982 meta-analysis by Peter Cohen, James Kulik, and Chen-Lin Kulik—published two years before Bloom’s essay but cited only half as often—reported that the average effect of tutoring was about 0.33 standard deviations, or 13 percentile points. Among 65 tutoring studies reviewed by the authors, only one (a randomized 1972 dissertation study that tutored 32 students) reported a two-sigma effect. More recently, a 2020 meta-analysis of randomized studies by Andre Nickow, Philip Oreopoulos, and Vincent Quan found that the average effect of tutoring was 0.37 standard deviations, or 14 percentile points—“impressive,” as the authors wrote, but far from two sigmas. Among 96 tutoring studies the authors reviewed, none produced a two-sigma effect.
So where did Bloom get the idea that the characteristic benefit of tutoring was two standard deviations? Was there anything behind Bloom’s two-sigma claim in 1984? Why are we still repeating it 40 years later?
What evidence did Bloom have?
Bloom’s Figure 1—reproduced in Khan’s TEDx talk, among many other places—ostensibly showed the distribution of post-test scores for students who received tutoring, comparing them to students who received conventional whole-group instruction and to students who received a version of what Bloom called “mastery learning,” which combined whole-group instruction with individualized feedback. But the graph was only illustrative—hand-drawn in a smooth, stylized fashion to show what a two-sigma effect might look like. It wasn’t fit to actual data.
Later in the essay, Bloom’s Table 1 compared the effects of different educational interventions. Tutoring appeared at the top of the list, with an effect of 2.00 standard deviations. Below tutoring, the table listed reinforcement learning (1.20 standard deviations), mastery learning (1.00 standard deviation) and a variety of other effects that seem startlingly large by modern standards.
Where did Bloom get these large, curiously round estimates? He claimed that he had adapted them from a paper summarizing early meta-analyses published a month earlier by Herb Walberg, a professor at the University of Illinois at Chicago. But Walberg’s and Bloom’s tables do not entirely agree (see Table 1). Although several of Bloom’s estimates lined up with Walberg’s, at least when rounded, most of the effects in Bloom’s table did not appear in Walberg’s, and most of the effects in Walberg’s table did not appear in Bloom’s. And the two professors definitely did not agree on the effect of tutoring.
Walberg didn’t put tutoring at the top of his list, and he estimated tutoring’s effect to be 0.40 standard deviations—close to the average effects reported in meta-analyses. Bloom did repeat Walberg’s estimate of 0.40 standard deviations, but he described it somewhat narrowly as the effect of “peer and cross-age remedial tutoring.” Walberg’s estimate wasn’t so circumscribed; he described it simply as the effect of tutoring.
Bloom relied on two students
Why did Bloom relabel Walberg’s tutoring effect of 0.40, and where did Bloom get his own estimate of 2.00? It seems Bloom was placing his faith in the dissertation studies of two of his PhD students, Joanne Anania and Arthur J. Burke. Both Anania and Burke reported two-sigma effects when comparing tutoring to whole-group classroom instruction—and substantial effects, though not as large, from mastery learning.
Because Anania and Burke provided essentially all the empirical evidence that backed Bloom’s claim of two-sigma tutoring, it’s a little shocking that Bloom didn’t credit them as coauthors. Bloom did cite his students’ dissertations, but if Burke and Anania had been coauthors on an instant classic like “The 2 Sigma Problem,” they might have gotten jobs that provided the resources to conduct further research on tutoring and mastery learning. Instead, Anania published a journal version of her dissertation research, which has been cited just 77 times to date. She taught at three universities in the Chicago area, where she specialized in reading, children’s literature, and adult literacy. Her 2012 obituary doesn’t mention her work on tutoring. Burke never published his dissertation research—or anything else on tutoring. Years later, he published half a dozen reports for the Northwest Regional Laboratory on suspension, expulsion, and graduation—not tutoring.
Bloom also did little work on tutoring after 1984. His next and last major project was an edited book titled Developing Talent in Young People. Published in 1985, the book relied on interviews with accomplished adults to reconstruct how they had developed their talents for music, sculpture, athletics, mathematics, or science. Bloom, who wrote only the introduction, summarized his two-sigma claim in a single paragraph that did not mention Anania or Burke. Bloom retired in 1991 and died in 1999.
It’s a little odd, isn’t it? If these three individuals—two of them just starting their research careers—really discovered a way to raise students’ test scores by two standard deviations, why didn’t they do more with it? Why didn’t they conduct more research? Why didn’t they start a tutoring company?
The two-sigma effect wasn’t just from tutoring
Did Anania and Burke really find two-sigma effects of tutoring? I must admit I was feeling skeptical when I printed out their dissertations. Few 40-year-old education findings hold up well, and student work, half of it unpublished, whose effects have never been replicated, seemed especially unpromising.
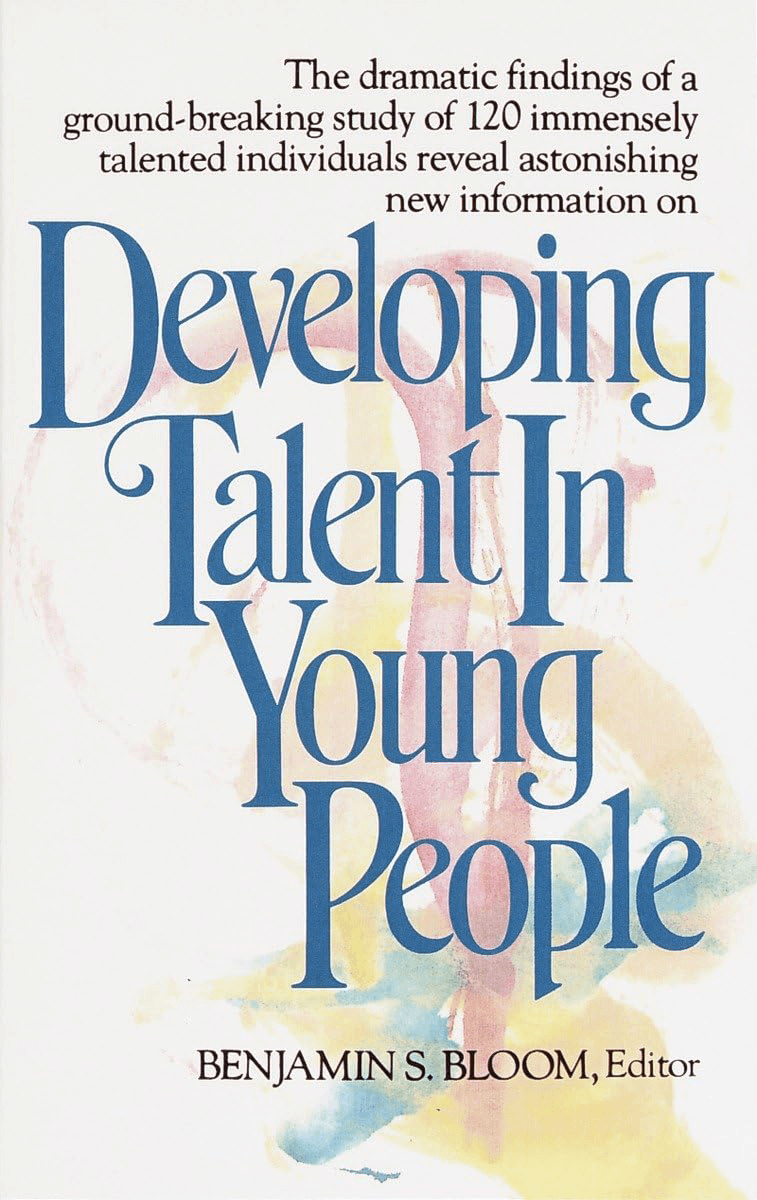
To my surprise, though, I found a lot to like in Anania’s and Burke’s dissertations. Both students ran small but nicely designed experiments to test the effect of a thoughtful educational intervention. They randomly assigned 4th, 5th, and 8th graders to receive whole-class instruction, mastery learning, or tutoring. The 4th and 5th graders learned probability; the 8th graders learned cartography. On a post-test given at the end of the three-week experiment, the tutored group really did outscore the whole-class group by two standard deviations on average.
But the tests that students took were very specific. And the tutoring intervention involved a lot more than just tutoring.
Students took a narrow test. Burke and Anania chose the topics of probability and cartography for a specific reason—because those topics were unfamiliar to participating students. There is nothing wrong with choosing an unfamiliar topic; experiments in the science of learning commonly do so. But it’s easier to produce a large effect when students are starting from zero. Cohen, Kulik, and Kulik’s 1982 meta-analysis reported that tutoring effects averaged 0.84 standard deviations when measured on narrow tests developed by the study authors, versus just 0.27 standard deviations when measured on broader standardized tests. In 2020, Matt Kraft reported that effects of educational interventions generally—not just tutoring—are about twice as large when they are evaluated based on narrow as opposed to broad tests.
While Anania’s and Burke’s intervention did achieve two-sigma effects on tests of the material covered in their three-week experiment, it is doubtful that they could achieve similar effects on a broad test like the SAT, which measures years of accumulated skills and knowledge, or on the state math and reading tests that so many parents and teachers have worried about since the pandemic.
Certainly not in three weeks.
Tutored students received extra testing and feedback. Burke’s and Anania’s two-sigma intervention did involve tutoring, but it also had other features. Perhaps the most important was that tutored students received extra testing and feedback. At the end of each unit, all students took a quiz, but any tutored student who scored below 80 percent (in Anania’s study) or 90 percent (in Burke’s) received feedback and correction on concepts that they had missed. Then the tutored students took a second quiz with new questions—a quiz that students in the whole-class condition never received. If the tutored students still scored below 80 or 90 percent, they got more feedback and another quiz.
Bloom acknowledged that his students’ experiments included extra quizzes and feedback, but he asserted that “the need for corrective work under tutoring is very small.” That assertion was incorrect. Clearly the tutored students benefited substantially from feedback and retesting (see Figure 2). For example, in week one of Anania’s experiment, tutored students scored 11 percentage points higher on the retest than they did on the initial test. In week two, tutored students scored 20 percentage points higher on the retest than on the initial test, and in week three, they scored 30 percentage points higher on the retest than on the initial test.
These boosts to performance, and their benefits for longer-term learning, are examples of the testing effect—an effect that, though widely appreciated in cognitive psychology today, was less appreciated in the 1980s. Students learn from testing and retesting, especially if they receive corrective feedback that focuses on processes and concepts instead of simply being told whether they are right or wrong. Burke’s and Anania’s tutors were trained on how to provide effective feedback. Indeed, Burke wrote, “perhaps the most important part of the tutors’ training was learning to manage feedback and correction effectively.” The feedback and retesting also provided tutored students with more instructional time than the students receiving whole-class instruction—about an hour more per week, according to Burke.
How much of the two-sigma effect did the extra testing and feedback explain? About half. You can tell because, in addition to the tutored and whole-class groups, there was a third group of students who engaged in “mastery learning,” which did not include tutoring but did include feedback and testing after whole-class instruction. On a post-test given at the end of the three-week experiment, the mastery-learning students scored about 1.1 standard deviations higher than the students who received whole-class instruction. That’s just a bit larger than the effects of 0.73 to 0.96 standard deviations reported by meta-analyses that have estimated the effects of testing and feedback on narrow tests.
If feedback and retesting accounted for 1.1 of Bloom’s two sigmas, that leaves 0.9 sigmas that we can chalk up to tutoring. That’s not too far from the 0.84 sigmas that the Cohen, Kulik, and Kulik meta-analysis reports for tutoring’s effect on narrow tests.
Tutors received extra training. Extra testing and feedback might have been the most important extra in Anania’s and Burke’s tutoring intervention, but it wasn’t the only extra.
Anania’s and Burke’s tutors also received training, coaching, and practice that other instructors in their experiments did not receive. Burke mentioned training tutors to provide effective feedback, but tutors were also trained “to develop skill in providing instructional cues . . . to summarize frequently, to take a step-by-step approach, and to provide sufficient examples for each new concept. . . . To encourage each student’s active participation, tutors were trained to ask leading questions, to elicit additional responses from the students, and to ask students for alternative examples or answers”—all examples of active, inquiry-based learning and retrieval practice. Finally, “tutors were urged to be appropriately generous with praise and encouragement whenever a student made progress. The purpose of this training was to help the tutor make learning a rewarding experience for each student.”
Although previous tutoring studies had not found larger effects if tutors were trained, the training these tutors received may have been exceptional. Anania and Burke could have isolated the effect of training if they had offered it to some of the instructors in the whole-class or mastery-learning group. Unfortunately, they didn’t do that, so we can’t tell how much of their tutoring effect was due to tutor training.
Tutoring was comprehensive. Many public and private programs offer tutoring as a supplement to classroom instruction. Students attend class with everyone else and then follow up with a tutor afterwards. But the tutoring in Burke’s and Anania’s experiments wasn’t like that. Tutoring didn’t supplement classroom instruction; tutoring replaced classroom instruction. Tutored students received all instruction from their tutors; they didn’t attend class at all. That’s important because, according to Cohen, Kulik, and Kulik’s meta-analysis, tutoring is about 50 percent more effective when it replaces rather than substitutes for classroom instruction.
It’s great, of course, that Burke’s and Anania’s students received the most effective form of tutoring. But it also means that it wasn’t the kind of tutoring that students commonly receive in an after-school or pull-out program.
All That Glitters
My father may have had a two-sigma tutor in 1945. His tutor couldn’t foresee Anania’s and Burke’s experiments, 40 years in the future, but her approach had several components in common with theirs. She met with her student frequently. She was goal-oriented, striving to ensure that my father mastered the 2nd- and 3rd-grade curricula rather than just putting in time. She didn’t yoke herself to the pace of classroom instruction but moved ahead as quickly as she thought my father could handle. And she checked his comprehension regularly—not with quizzes but with short homework assignments, which she checked and corrected to explain his mistakes.
But not all tutoring is like that, and some of what passes for tutoring today is much worse than what my father received in 1945.
In the fall of 2020, I learned that my 5th grader’s math scores had declined during the pandemic. I knew that they hadn’t been learning much math, but the fact that their skills had gone backward was a bit of a shock.
To prepare them for what would come next, I told them the story about my father’s 2nd-grade tutor.
“Grandpa got tutored every day for seven weeks?” they asked me. “That seems excessive.”
“You think so?” I asked.
“Yeah—it’s 47 hours!”
“Come again?” I asked.
They reached for a calculator.
Once a week I drove them to a for-profit tutoring center at a nearby strip mall. It was a great time to be in the tutoring business, but this center wasn’t doing great things with the opportunity. My child sat with four other children, filling out worksheets while a lone tutor sat nearby—available for questions, but mostly doing her own college homework and exchanging text messages with her friends. One day my child told me that they had spent the whole hour just multiplying different numbers by eight. They received no homework. From a cognitive-science perspective, I was pretty sure that practicing a single micro-skill for an hour once a week was not optimal. The whole system seemed designed not to catch kids up, but to keep parents coming back and paying for sessions.
Unfortunately, overpriced and perfunctory tutoring is common. In an evaluation of private tutoring services purchased for disadvantaged students by four large school districts in 2008–2012, Carolyn Heinrich and her colleagues found that, even though districts paid $1,100 to $2,000 per eligible student (40 percent more in current dollars), students got only half an hour each week with a tutor, on average. Because districts were paying per student instead of per tutor, most tutors worked with several children at once, providing little individualized instruction, even for children with special needs or limited English. Students met with tutors outside of regular school hours, and student engagement and attendance were patchy.
Only one district—Chicago—saw positive impacts of tutoring, and those impacts averaged just 0.06 standard deviations, or 2 percentile points.
My grandmother would never have stood for that.
After these results were published, some of Chicago’s most disadvantaged high schools started working with a new provider, Saga Education. Compared to the tutoring services that Heinrich and her colleagues evaluated, Saga’s approach was much more structured and intense. Tutors were trained for 100 hours before starting the school year. They worked with just two students at a time. Tutoring was scheduled like a regular class, so that students met with their tutor for 45 minutes a day, and the way the tutor handled that time was highly regimented. Each tutoring session began with warmup problems, continued with tutoring tailored to each student’s needs, and ended with a short quiz.
The cost of Saga tutoring—$3,500 to $4,300 per student per year—was higher than the programs that Heinrich and her colleagues had evaluated, but the results were much better. According to a 2021 evaluation by Jonathan Guryan and his colleagues, Saga tutoring raised math scores by 0.16 to 0.37 standard deviations. The effect was “sizable,” the authors concluded—it wasn’t two sigmas, but it doubled or even tripled students’ annual gains in math.
Is Two-Sigma Tutoring Real?
The idea that tutoring consistently raises achievement by two standard deviations is exaggerated and oversimplified. The benefits of tutoring depend on how much individualized instruction and feedback students get, how much they practice the tutored skills, and on the type of test used to measure tutoring’s effects. Tutoring effects, as estimated by rigorous evaluations, have ranged from two full standard deviations down to zero or worse. About one-third of a standard deviation seems to be the typical effect of an intense, well-designed program evaluated against broad tests.
The two-sigma effects obtained in the 1980s by Anania and Burke were real and remarkable, but they were obtained on a narrow, specialized test, and they weren’t obtained by tutoring alone. Instead, Anania and Burke mixed a potent cocktail of interventions that included tutoring; training and coaching in effective instructional practices; extra time; and frequent testing, feedback, and retesting.
In short, Bloom’s two-sigma claim had some basis in fact, but it also contained elements of fiction.
Like some science fiction, though, Bloom’s claim has inspired a great deal of real progress in research and technology. Modern cognitive tutoring software, such as ASSISTments or MATHia, was inspired in part by Bloom’s challenge, although what tutoring software exploits even more is the feedback and retesting required for mastery learning. Video tutoring makes human tutors more accessible, and new chatbots have the potential to make AI tutoring almost as personal, engaging, and responsive. Chatbots are also far more available and less expensive than human tutors. Khanmigo, for example, costs $9 a month, or $99 per year.
My own experience suggests that the large language models that undergird AI tutoring, by themselves, quickly get lost when trying to teach common math concepts like the Pythagorean theorem. But combining chatbots’ natural language capabilities with a reliable formal knowledge base—such as a cognitive tutor, a math engine, or an open-source textbook—offers substantial promise.
There is also the question of how well students will engage with a chatbot. Since chatbots aren’t human, it is easy to imagine that students won’t take them seriously—that they won’t feel as accountable to them as my father felt to his tutor and his mother. Yet students do engage and even open up to chatbots, perhaps because they know they won’t be judged. The most popular chatbots among young people are ones that simulate psychotherapy. How different is tutoring, really?
It seems rash, though, to promise two-sigma effects from AI when human tutoring has rarely produced such large effects, and no evidence on the effects of chatbot tutoring has yet been published. Over-promising can lead to disappointment, and reaching for impossible goals can breed questionable educational practices. There are already both human and AI services that will do students’ homework for them, as well as more well-intentioned but still “overly helpful” tutors who help students complete assignments without fully understanding what they’re doing. Such tutors may raise students’ grades in the short term, but in the long run they cheat students of the benefits of learning for themselves.
In the early going, it would be sensible simply to aim for effects that approximate the benefits of well-designed human tutoring. Producing benefits of one-third of a standard deviation would be a huge triumph if it could be done at low cost, on a large scale, and on a broad test—all without requiring an army of human tutors, some of whom may not be that invested in the job. Effects of one-third of a standard deviation probably won’t be achieved just by setting chatbots loose in the classroom but might be within reach if we skillfully integrate the new chatbots with resources and strategies from the science of learning. Once effects of one-third of a standard deviation have been produced and verified, we should be able to improve on them through continuous, incremental A/B testing—slowly turning science fiction into science fact.
Paul von Hippel is a professor and associate dean for research in the LBJ School of Public Affairs at the University of Texas, Austin.
This article appeared in the Spring 2024 issue of Education Next. Suggested citation format:
von Hippel, P.T. (2024). Two-Sigma Tutoring: Separating Science Fiction from Science Fact. Education Next, 24(2), 22-31.
For more, please see “The Top 20 Education Next Articles of 2024.”